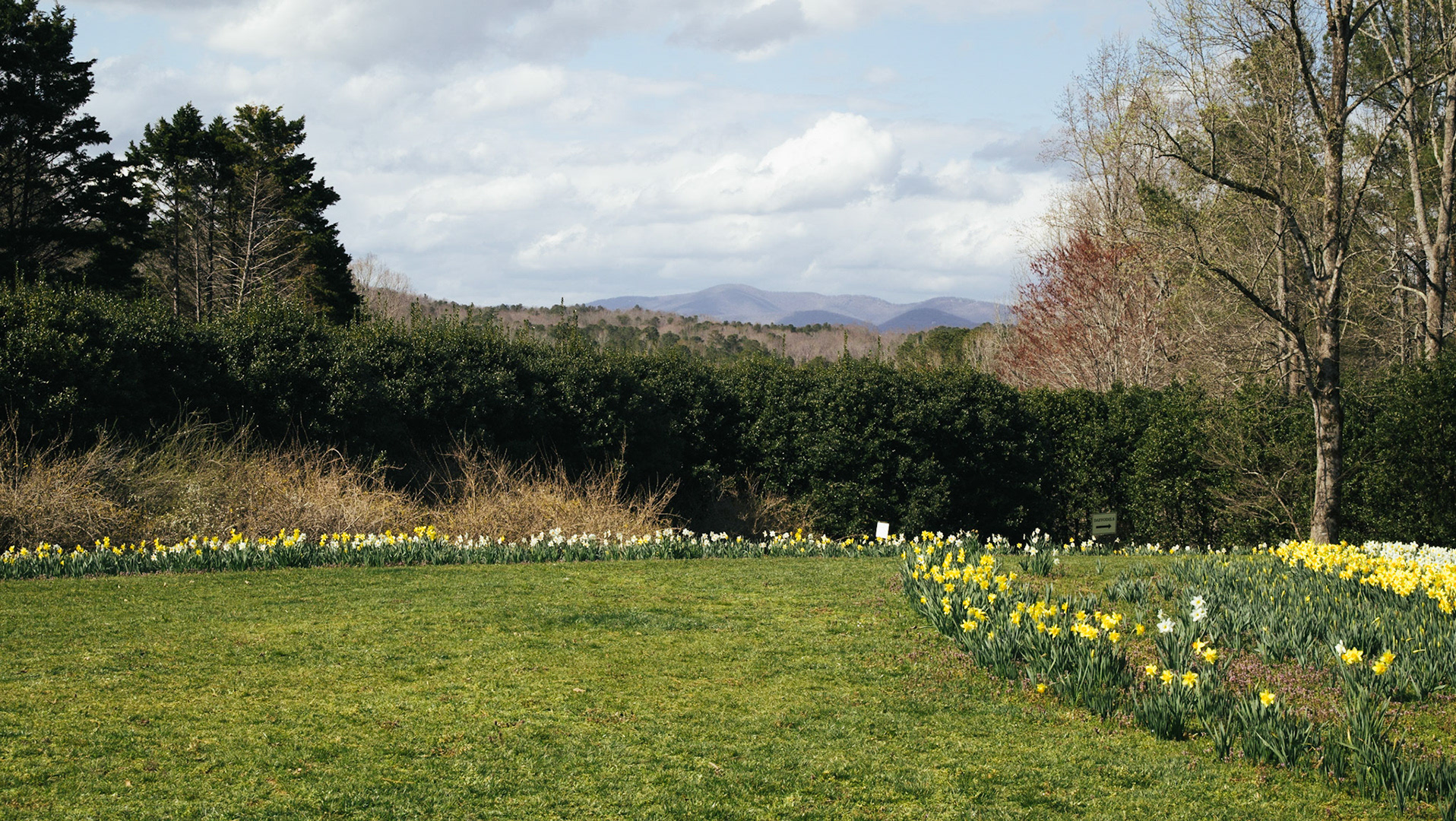
Gibbs Gardens
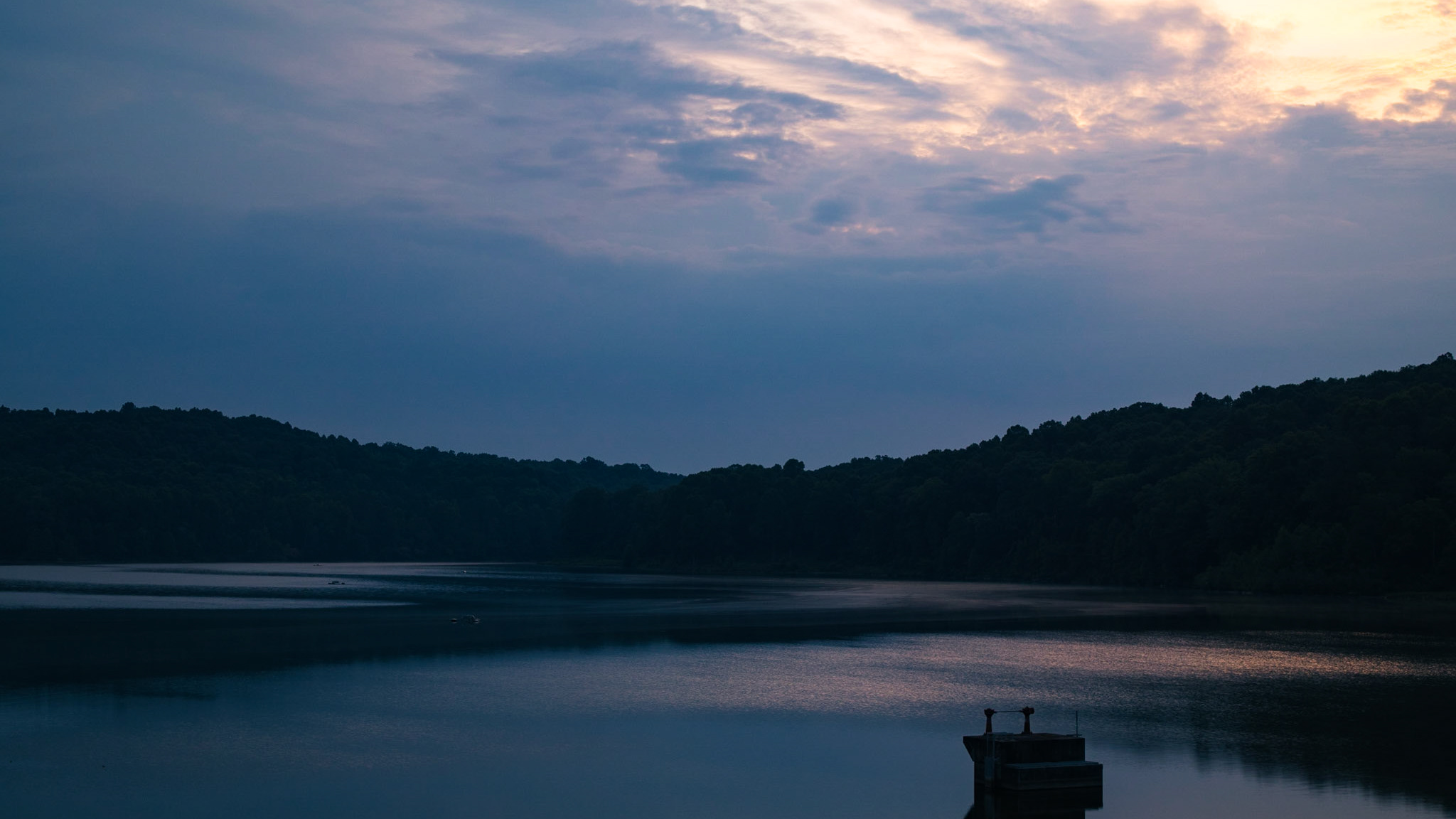
Lake Zwerner
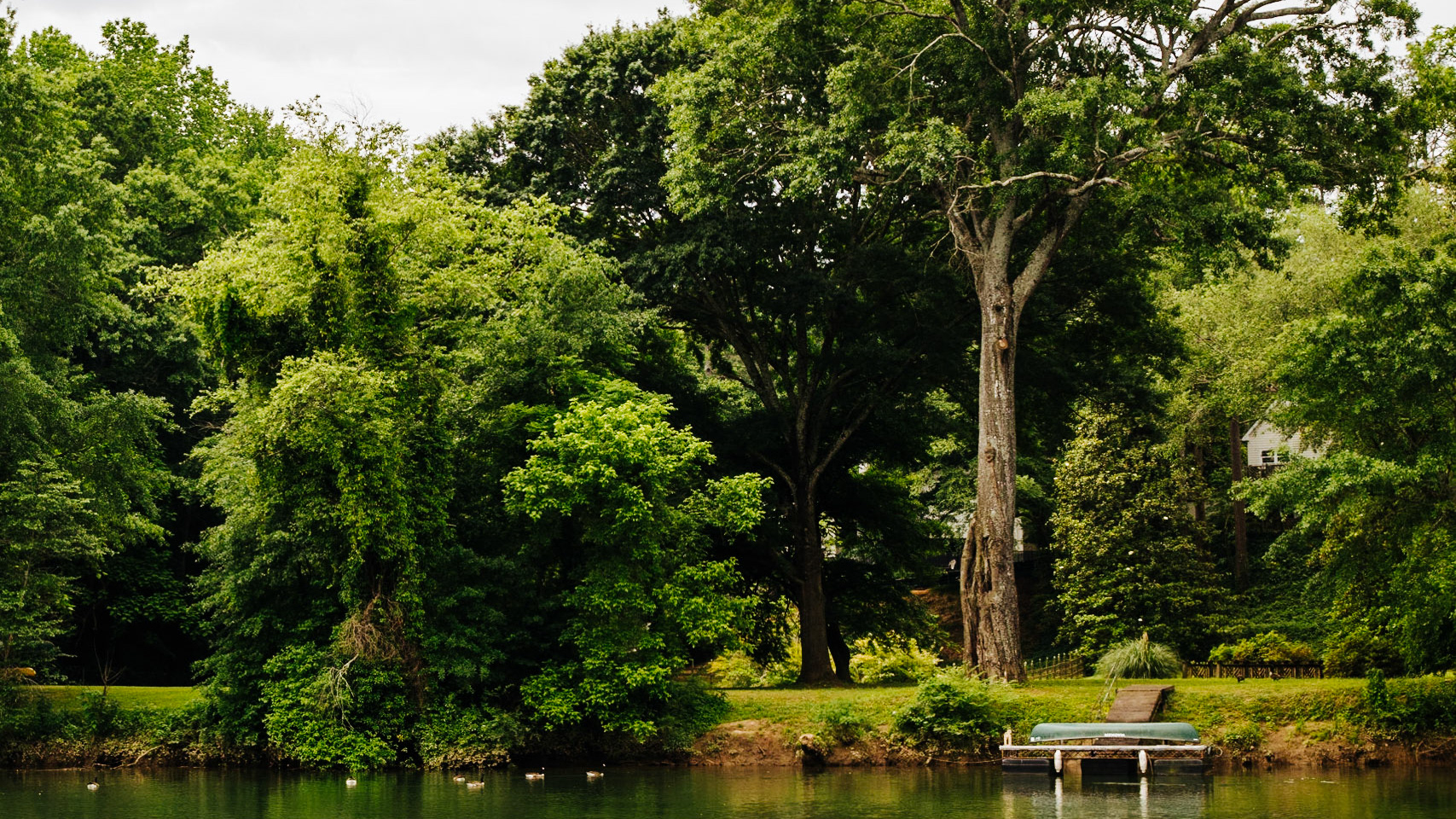
Chattahoochee
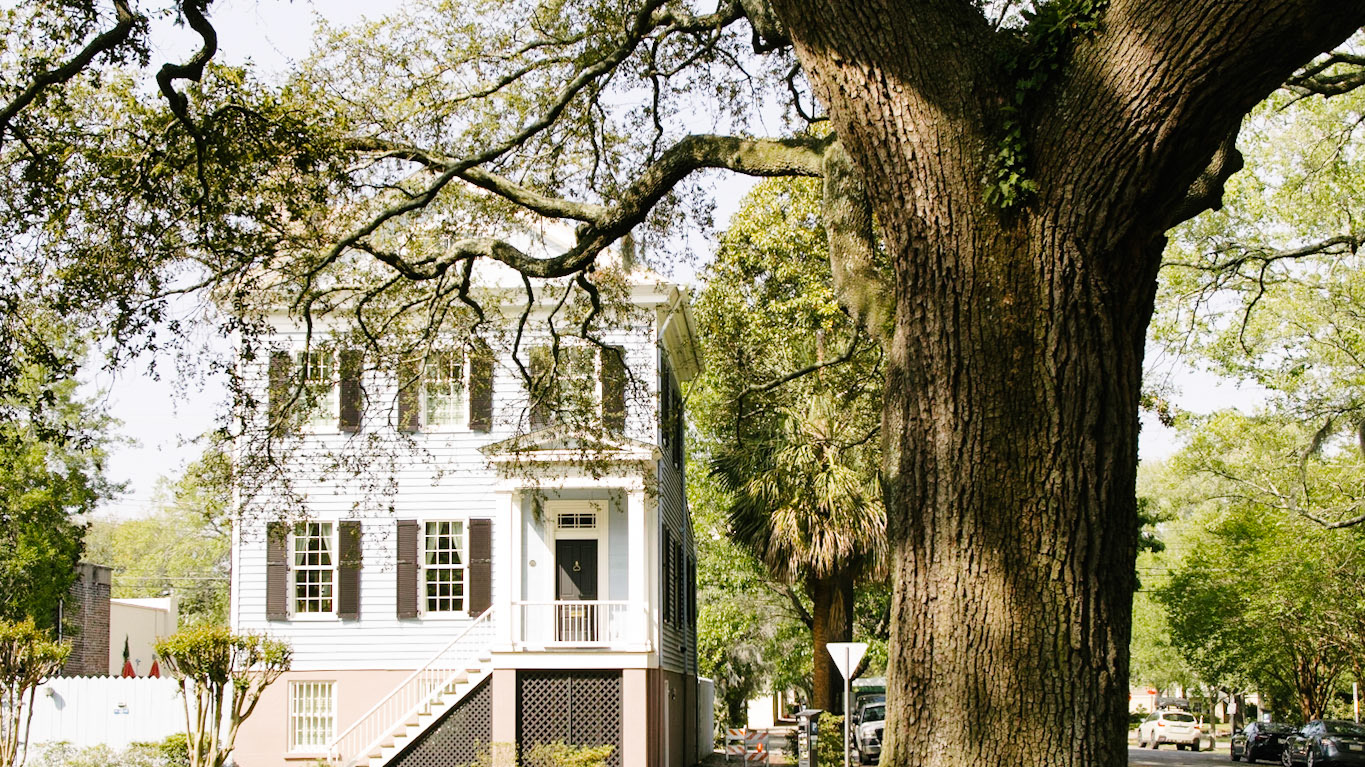
Savannah
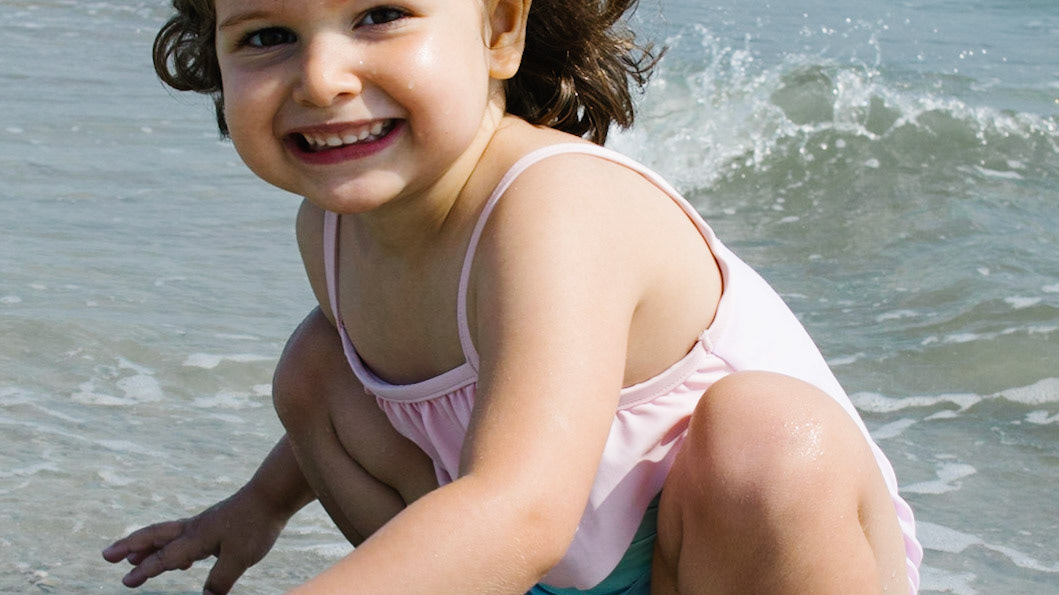
Faces
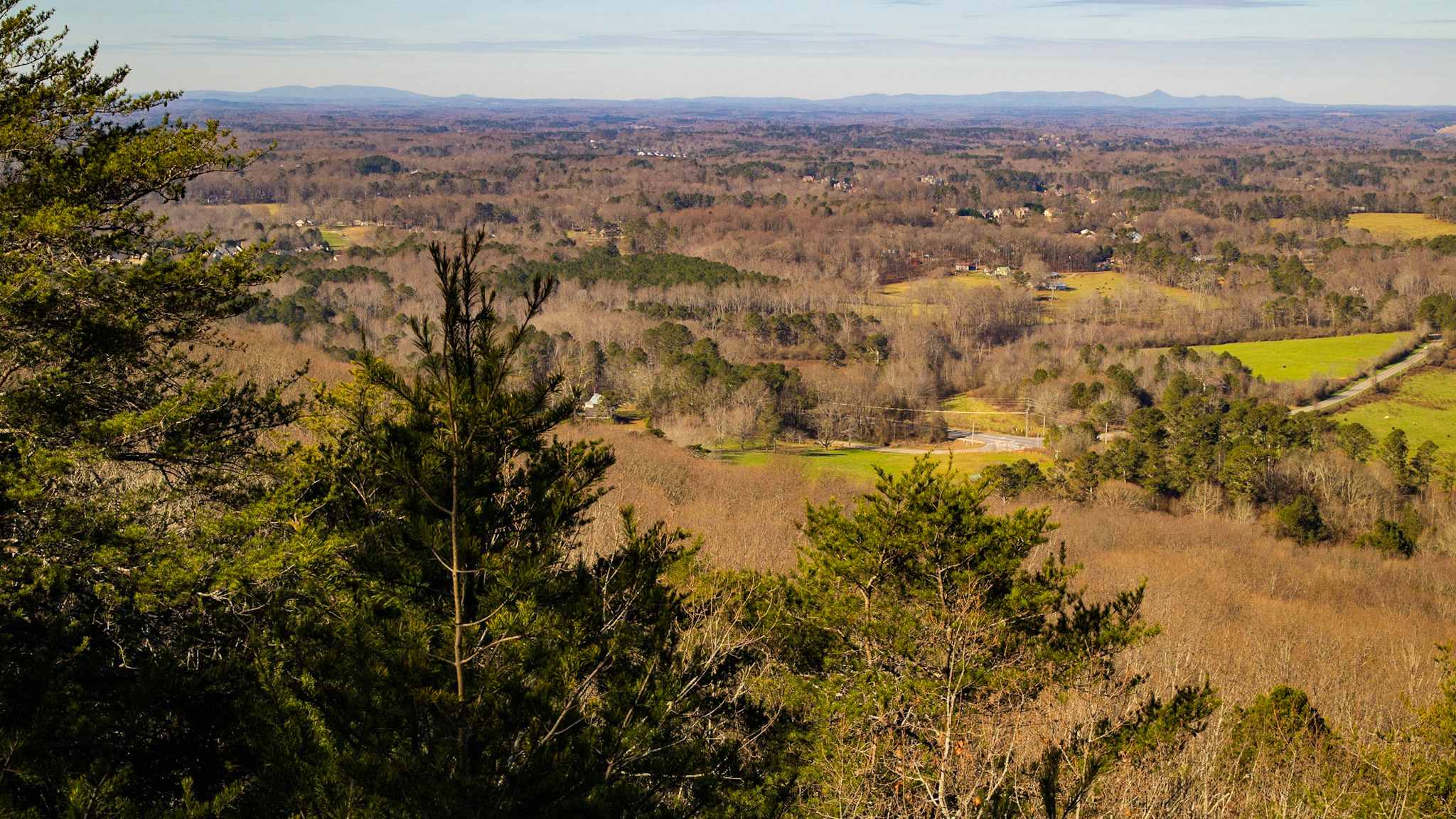
Sawnee Mountain
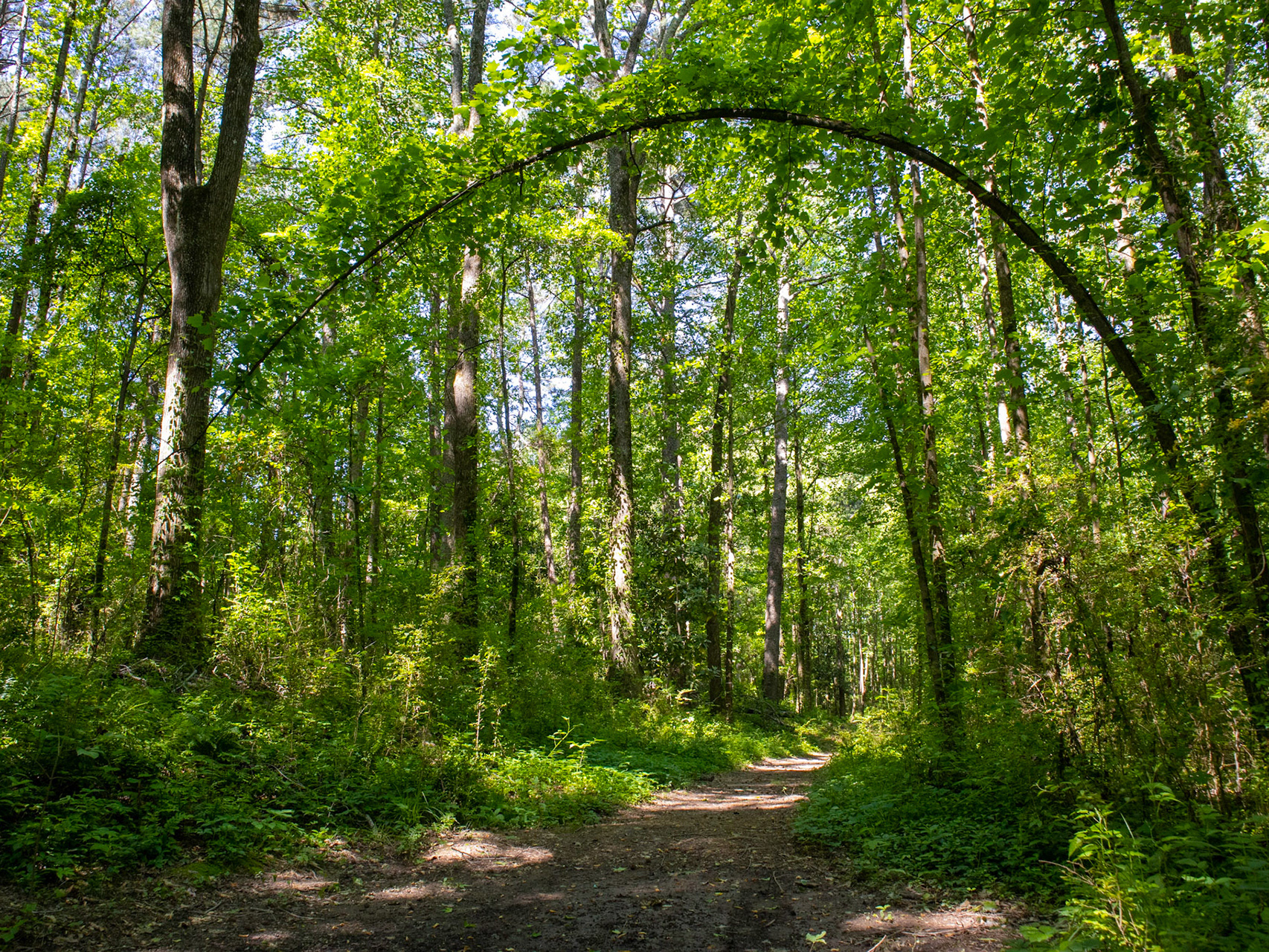
Wills Park
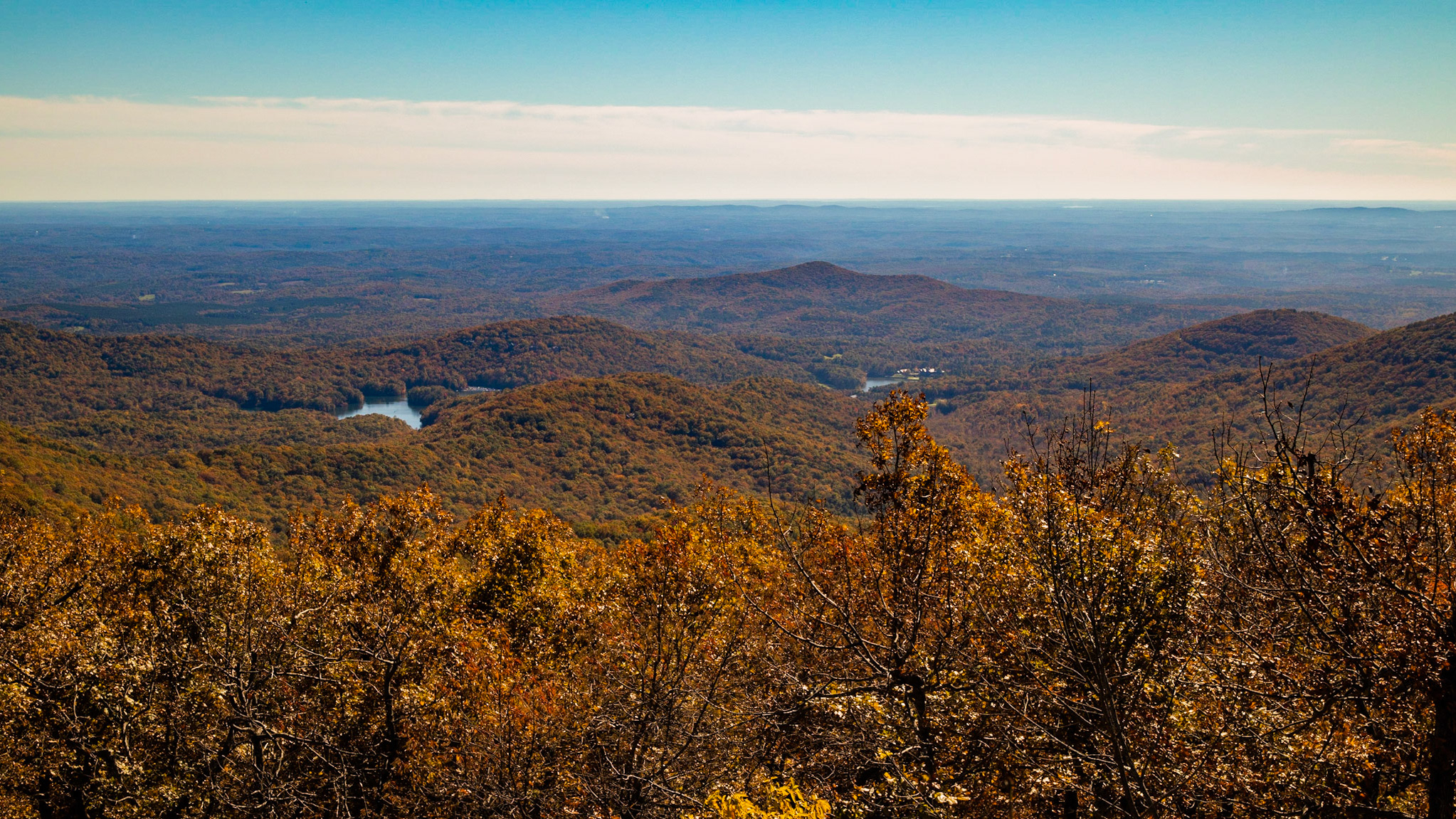
Eagle's Rest
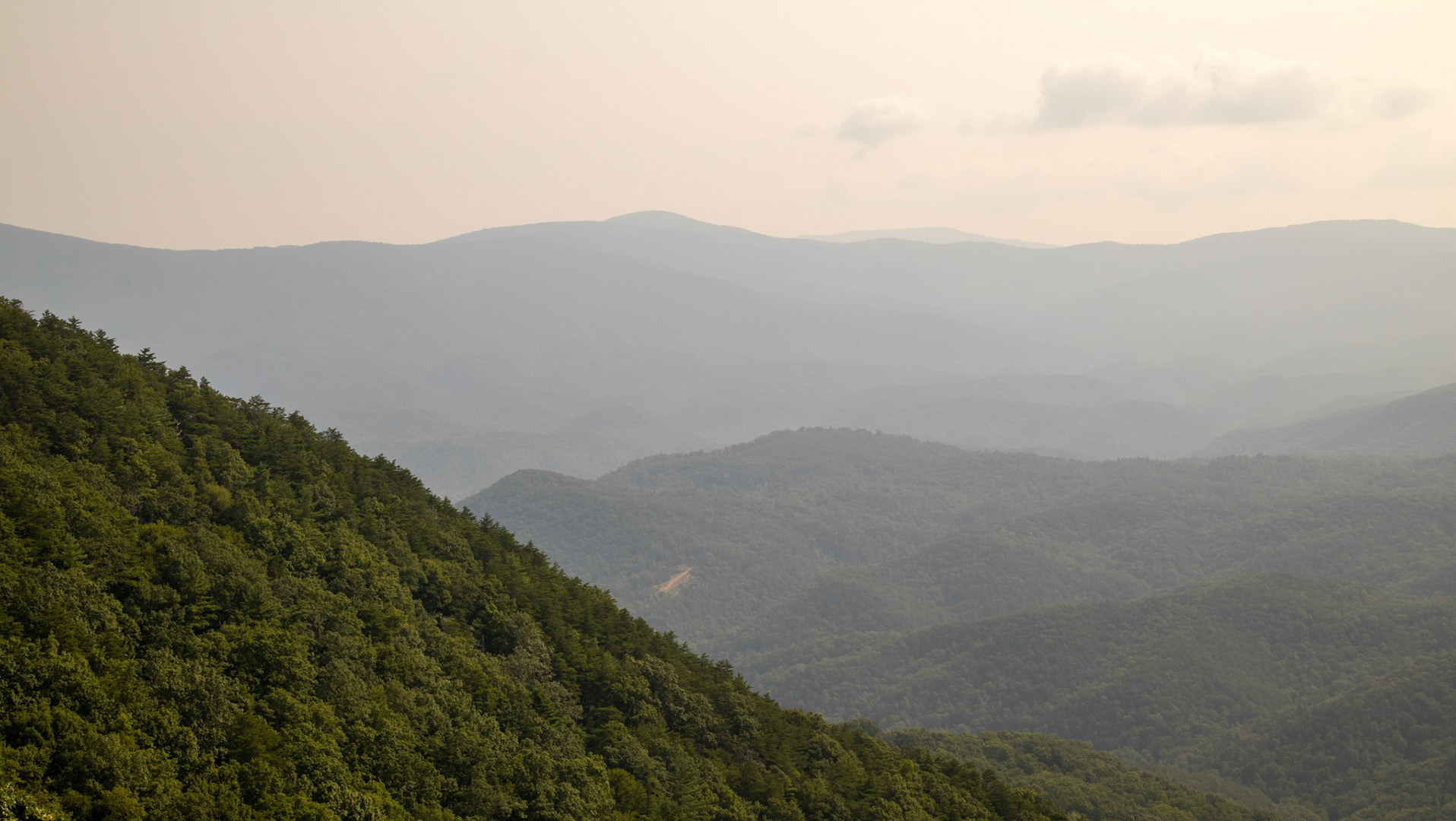
Fort Mountain
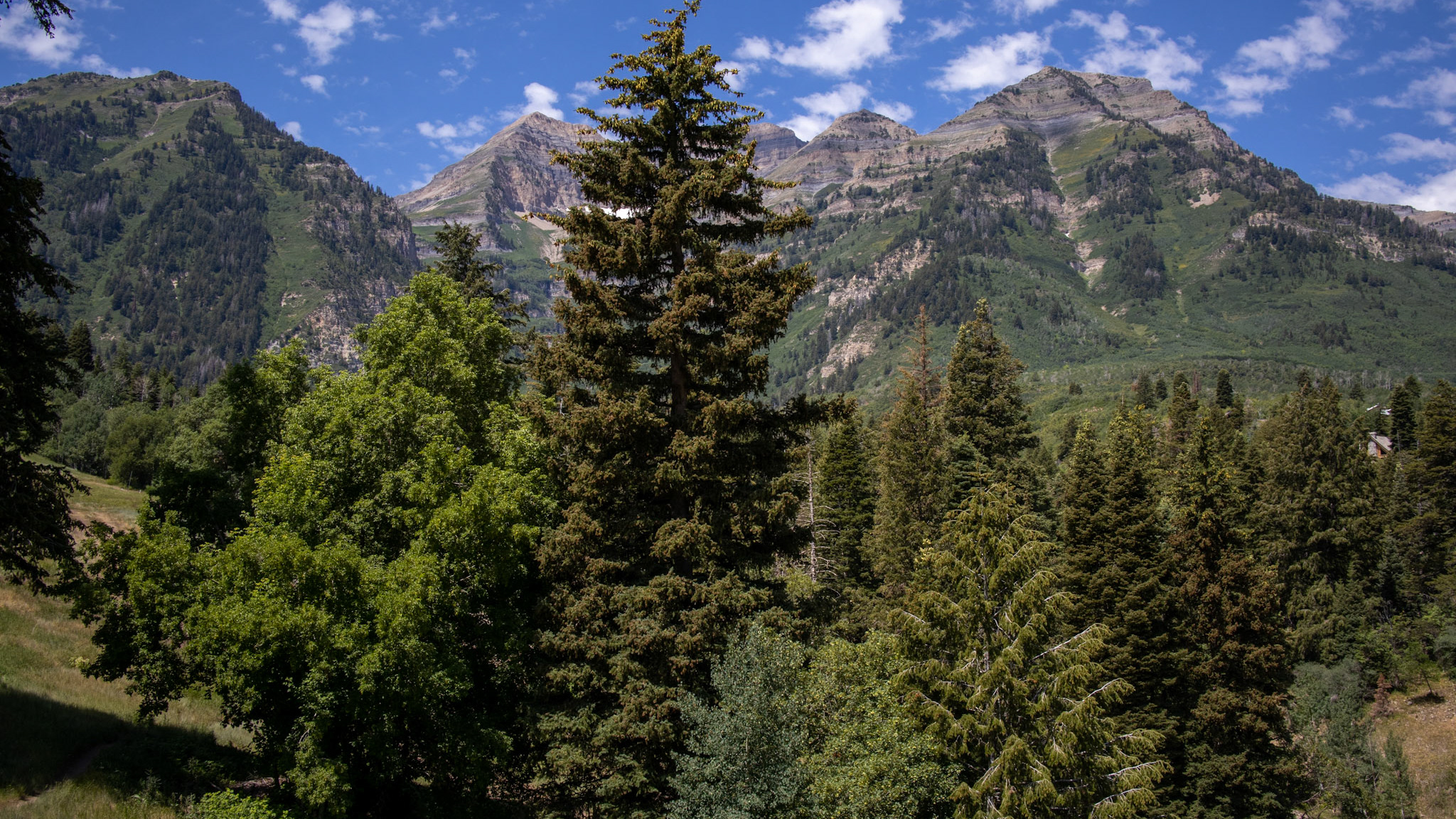
Sundance
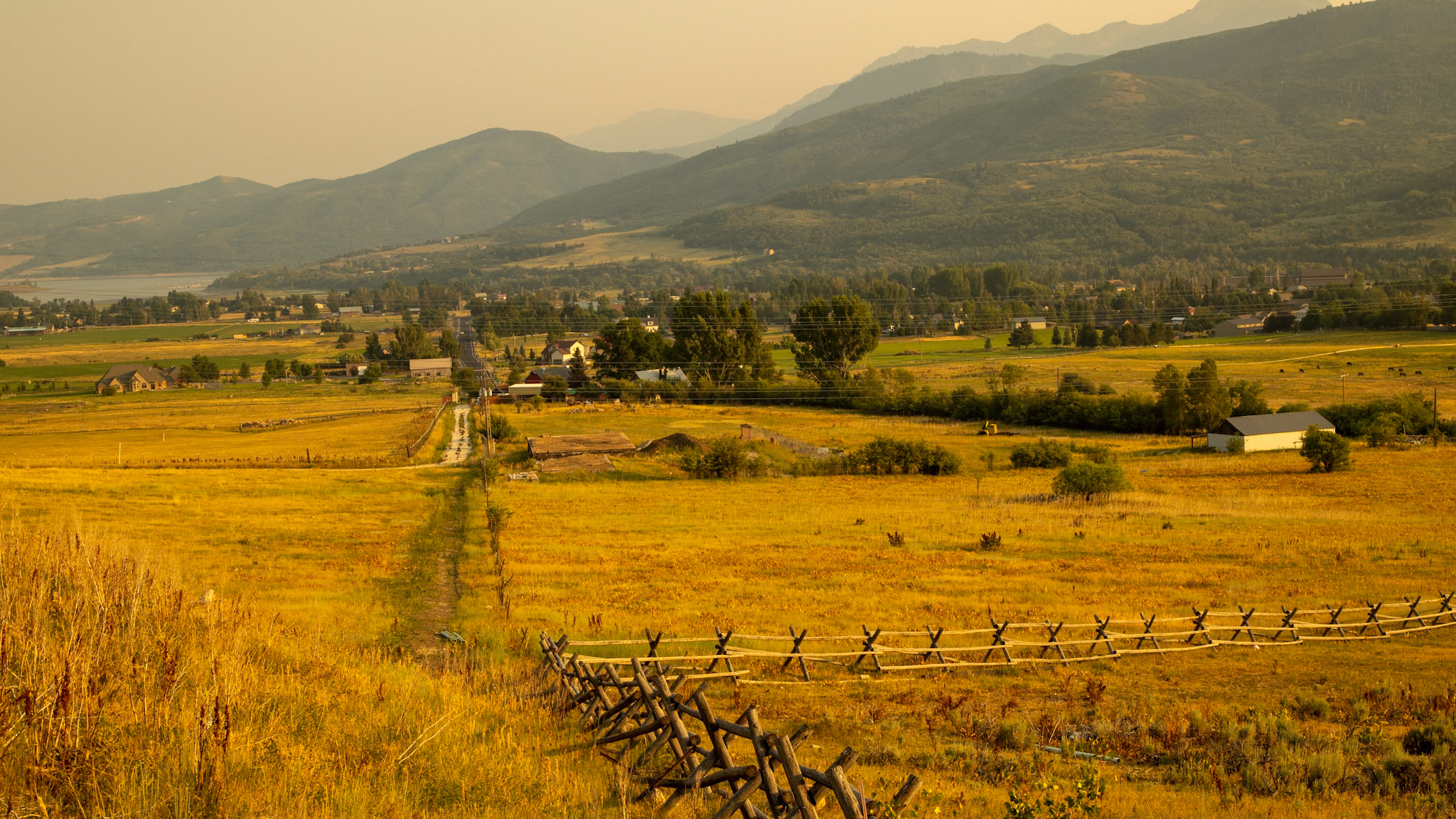
Eden Utah
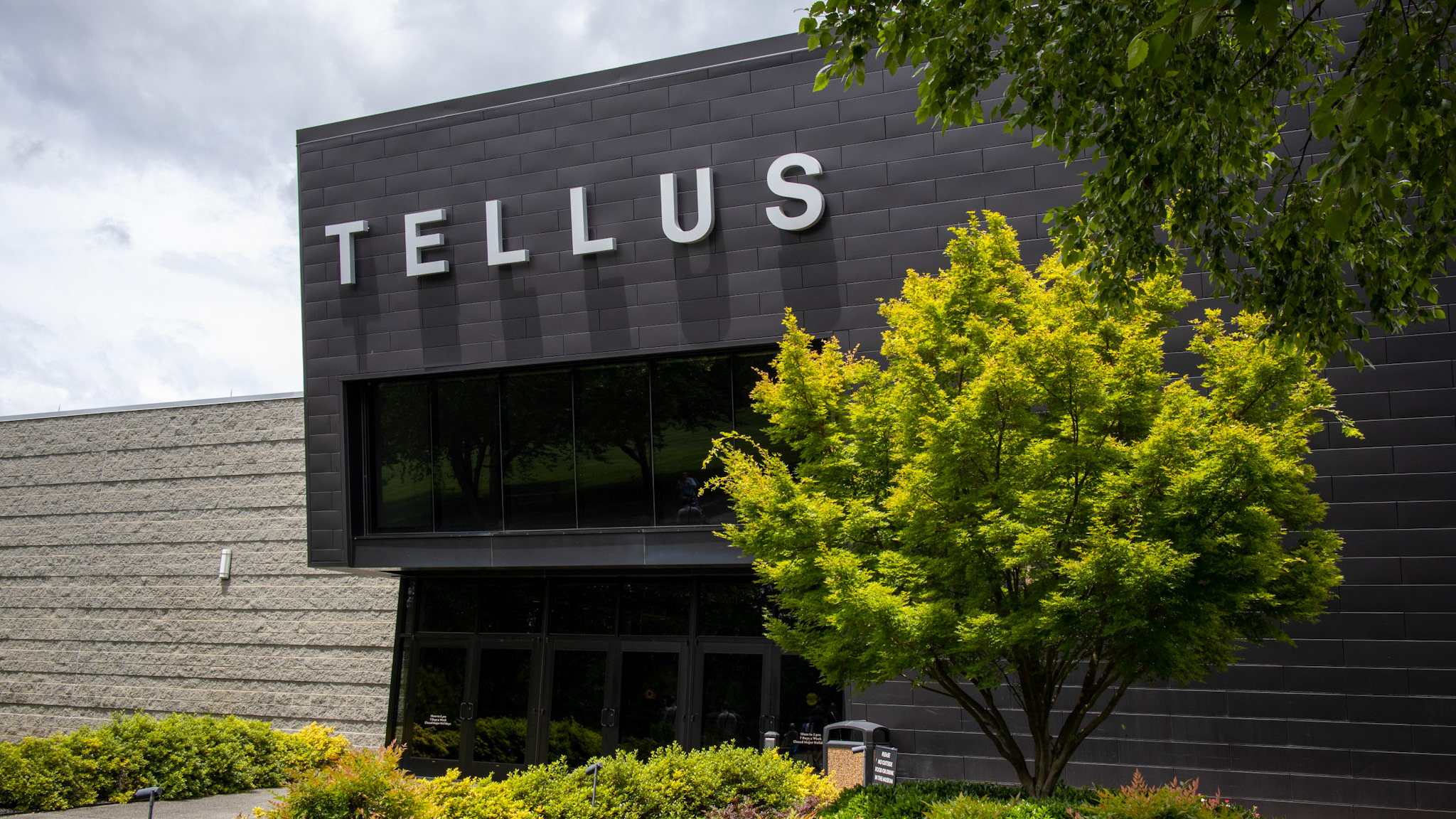
Tellus
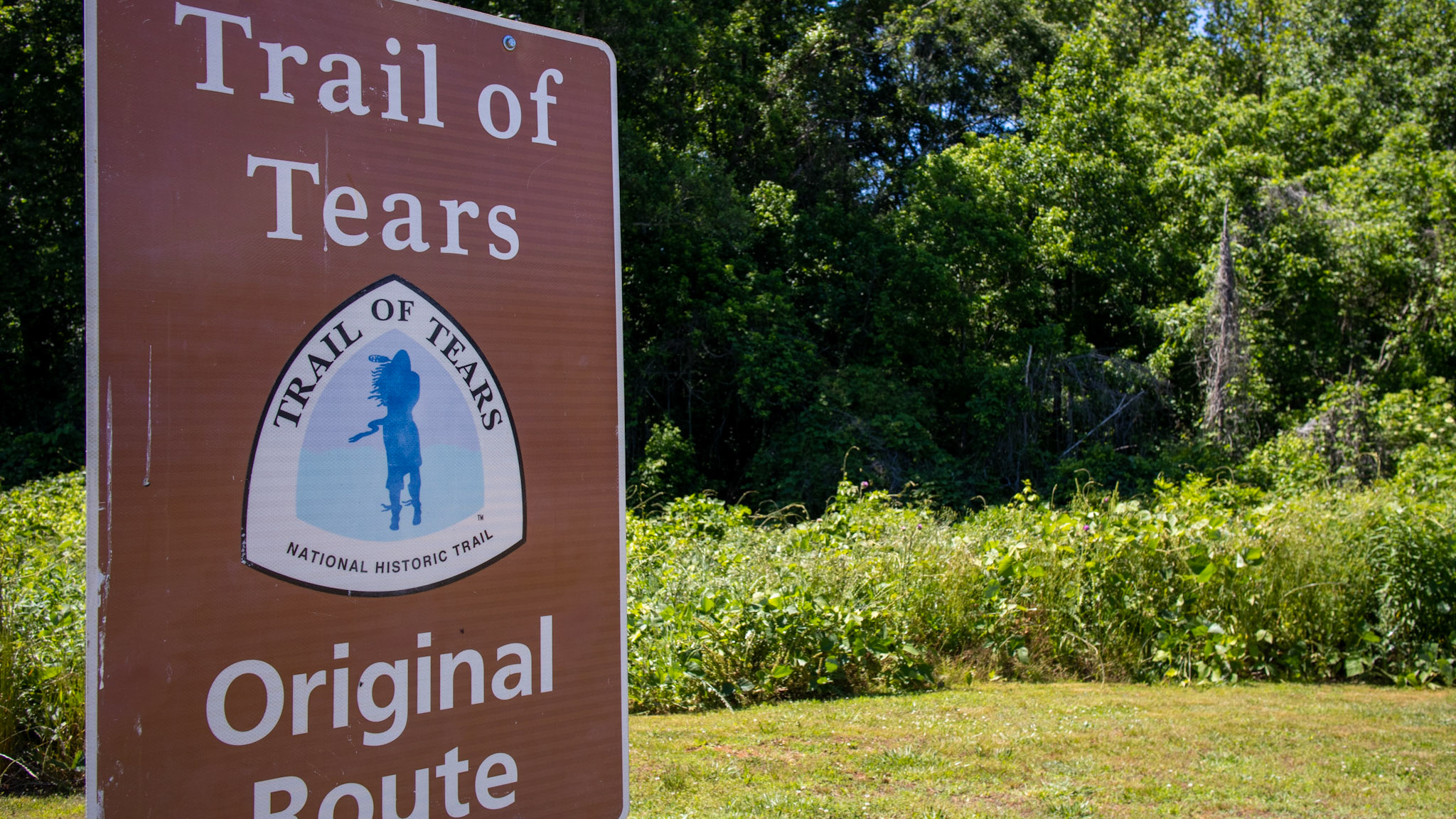
Trail of Tears
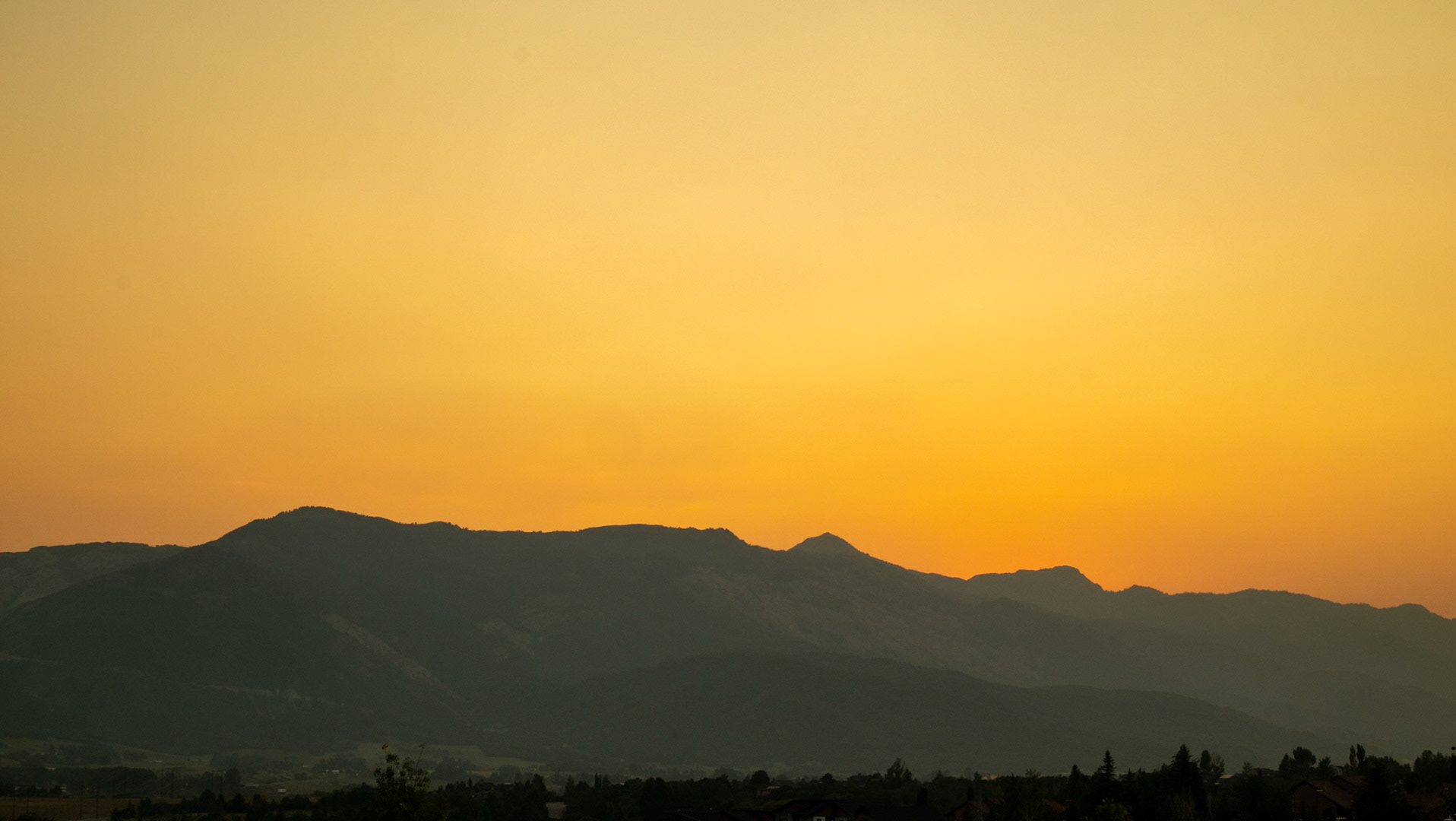
Sunrise Sunset
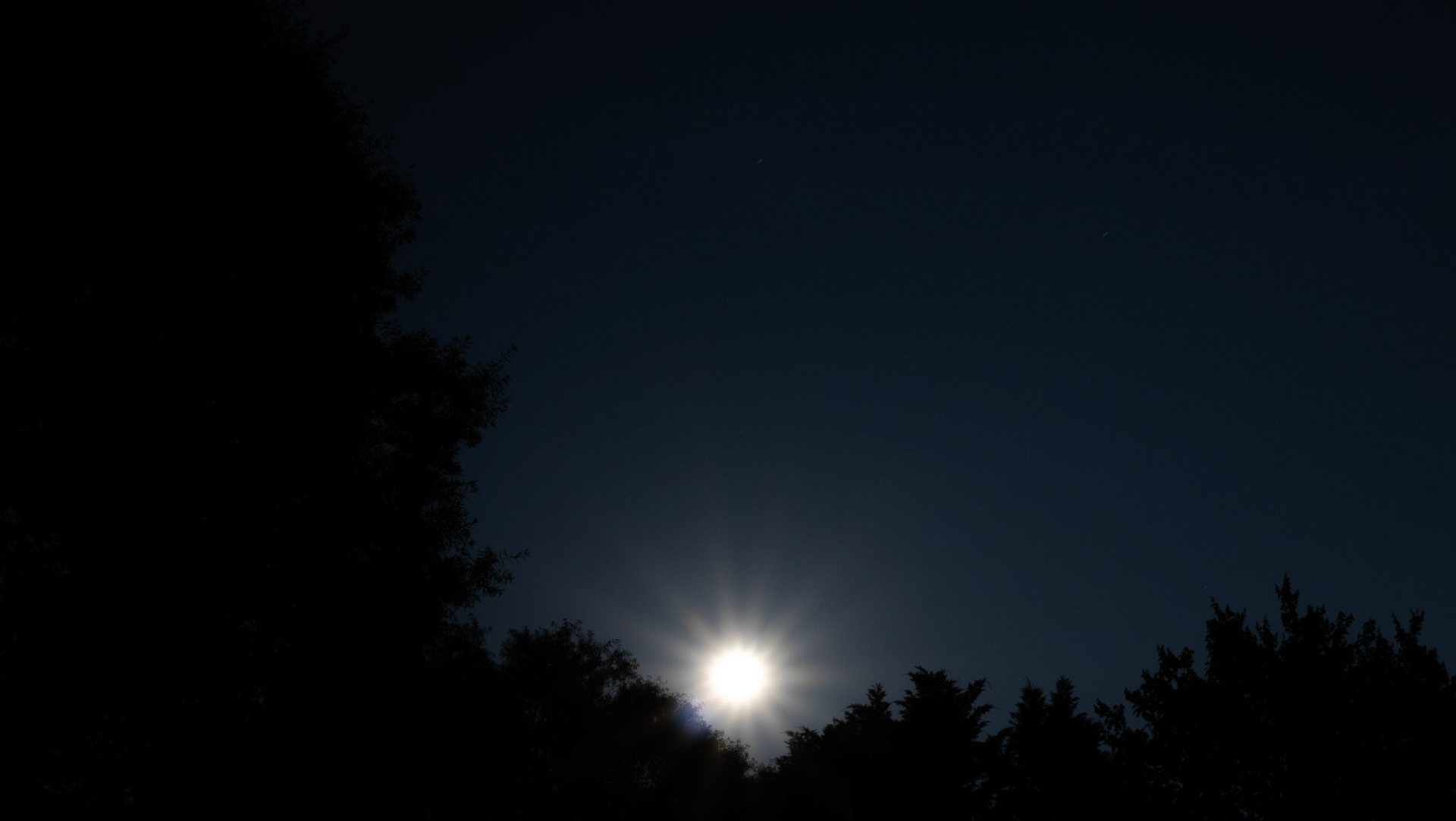
Night
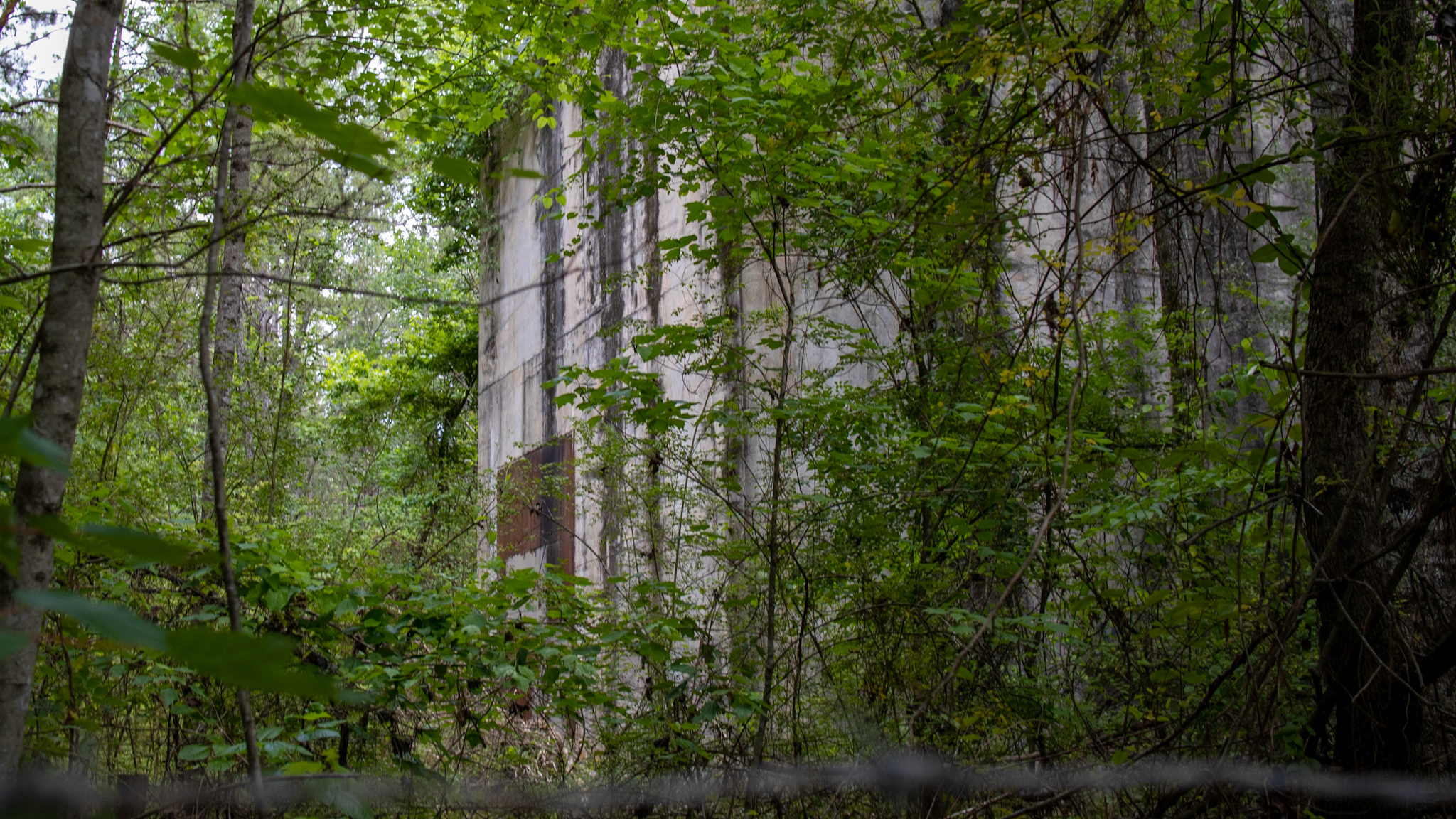
Nuclear Aircraft Facility
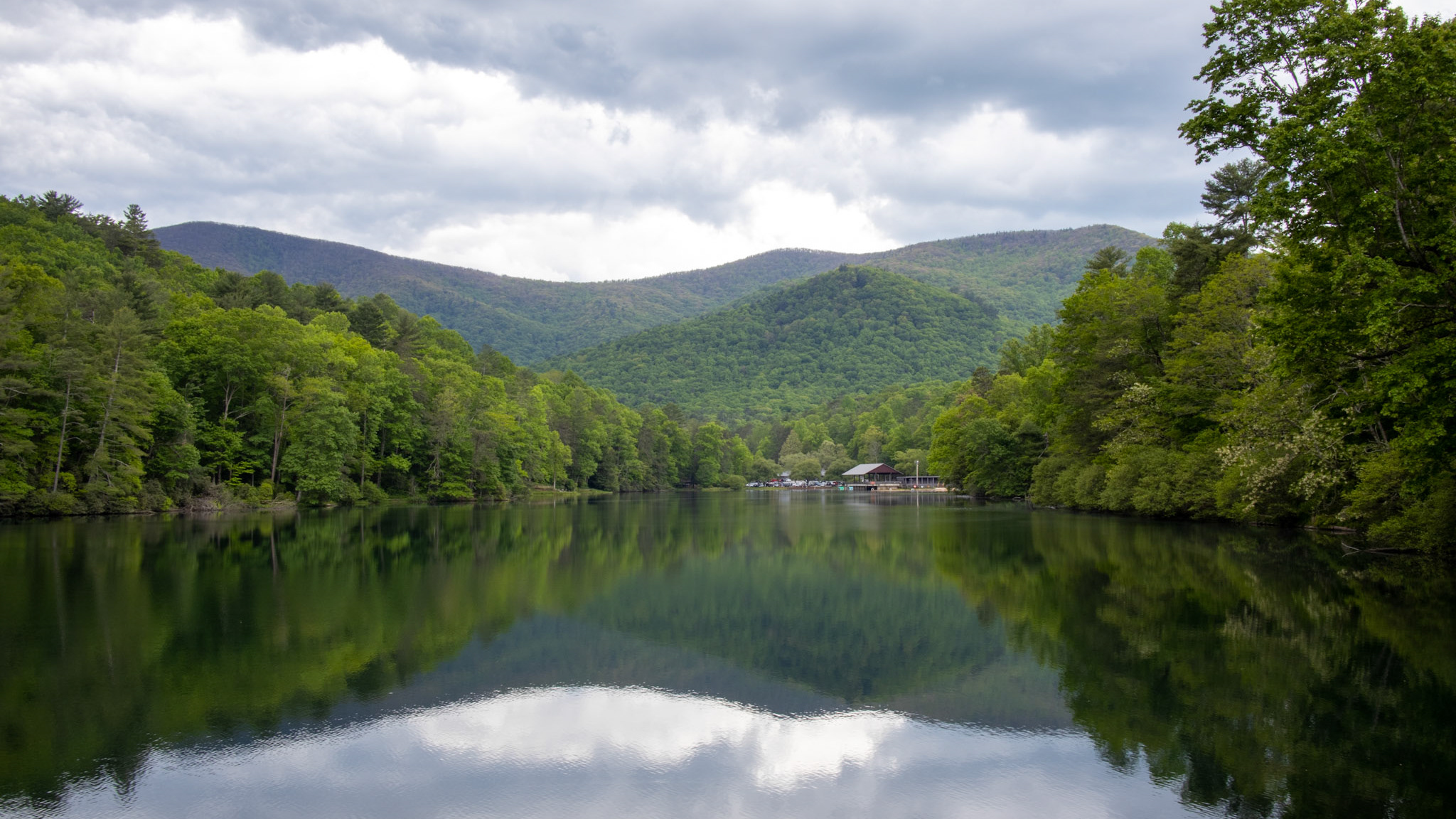
Vogel State Park
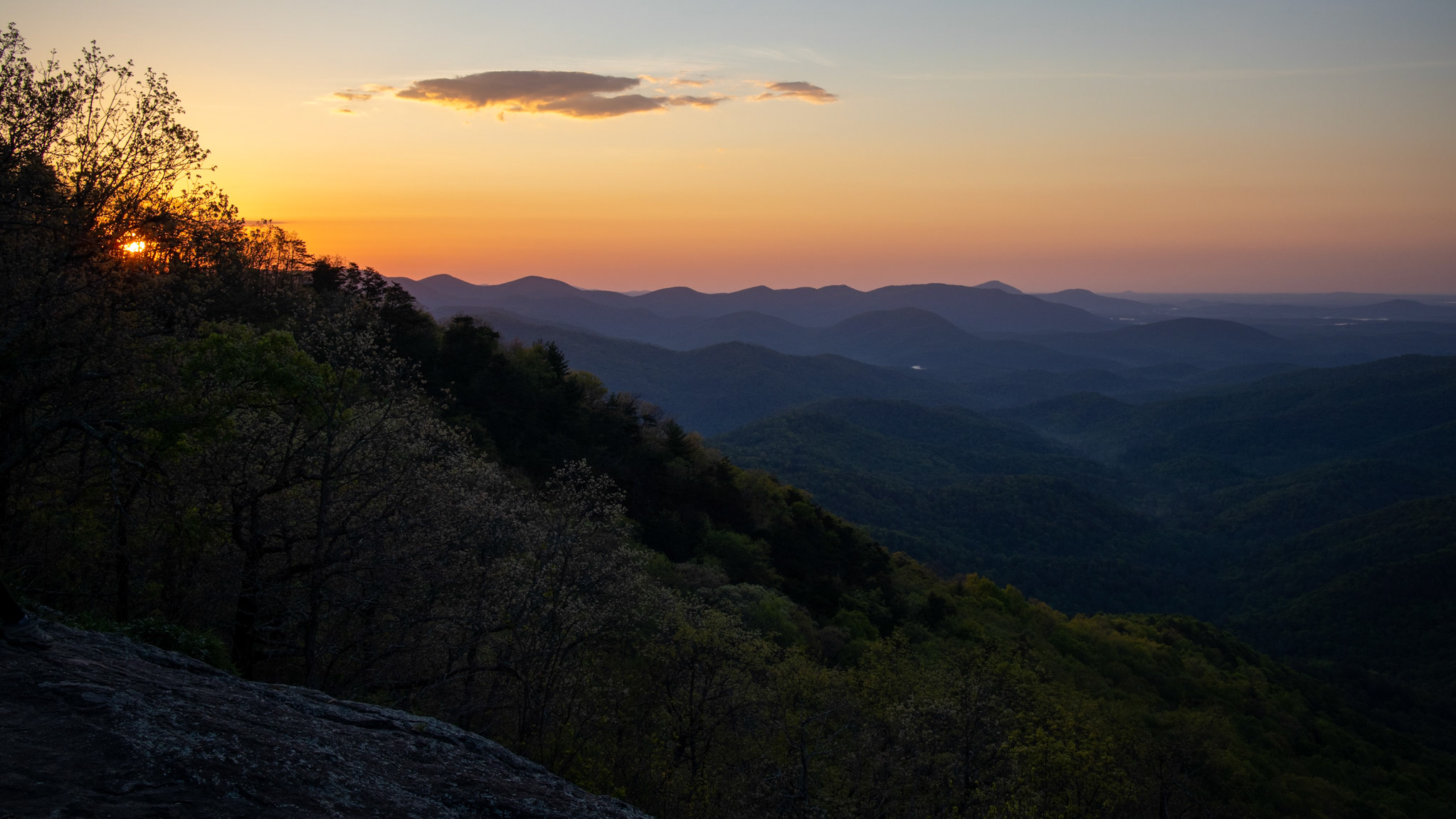
Preacher's Rock
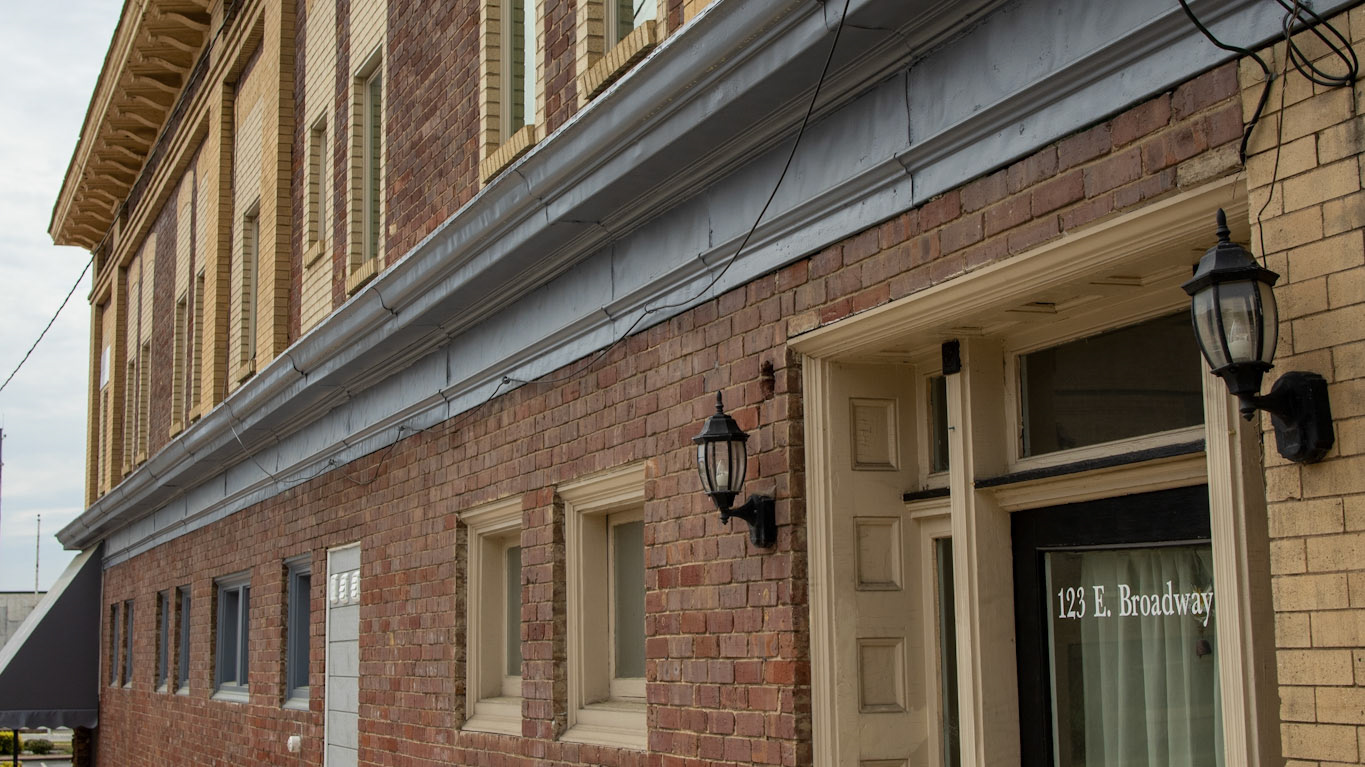
Lenoir City
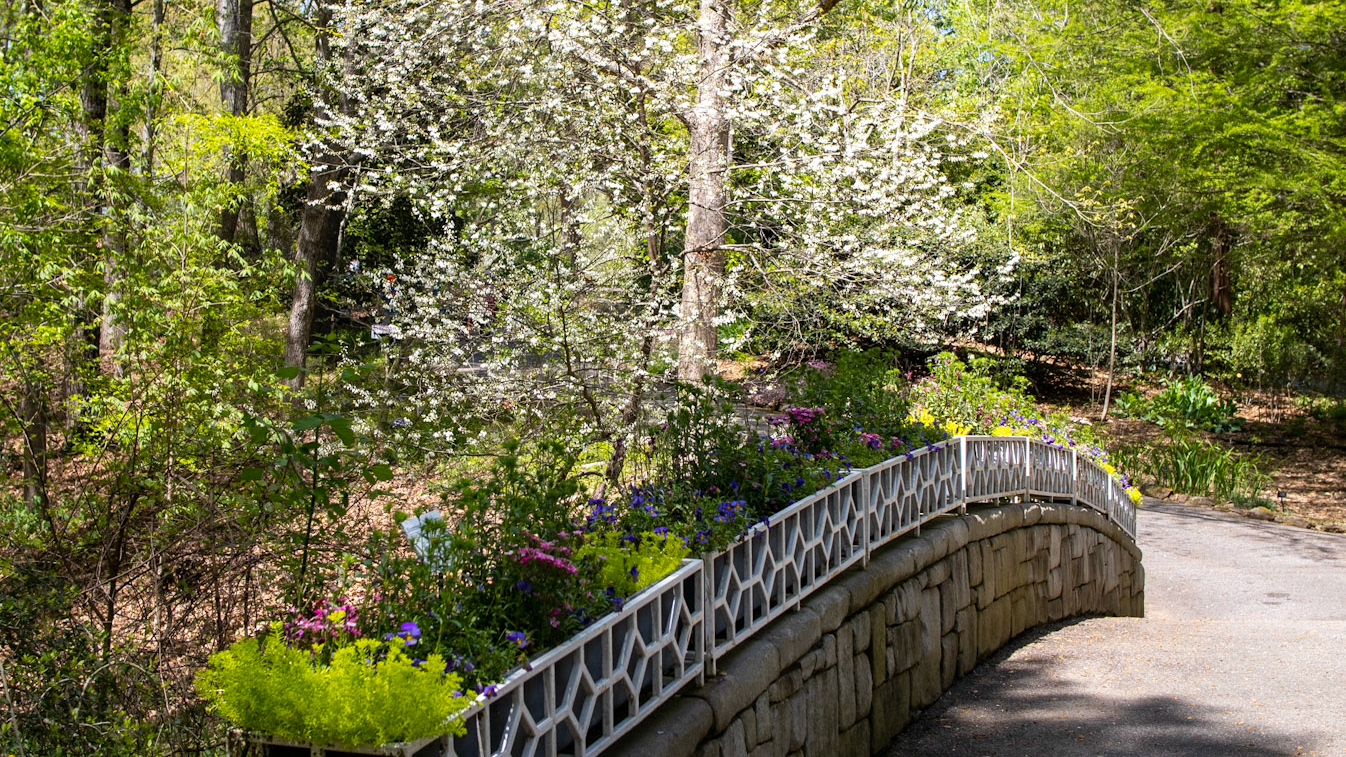
State Botanical Garden
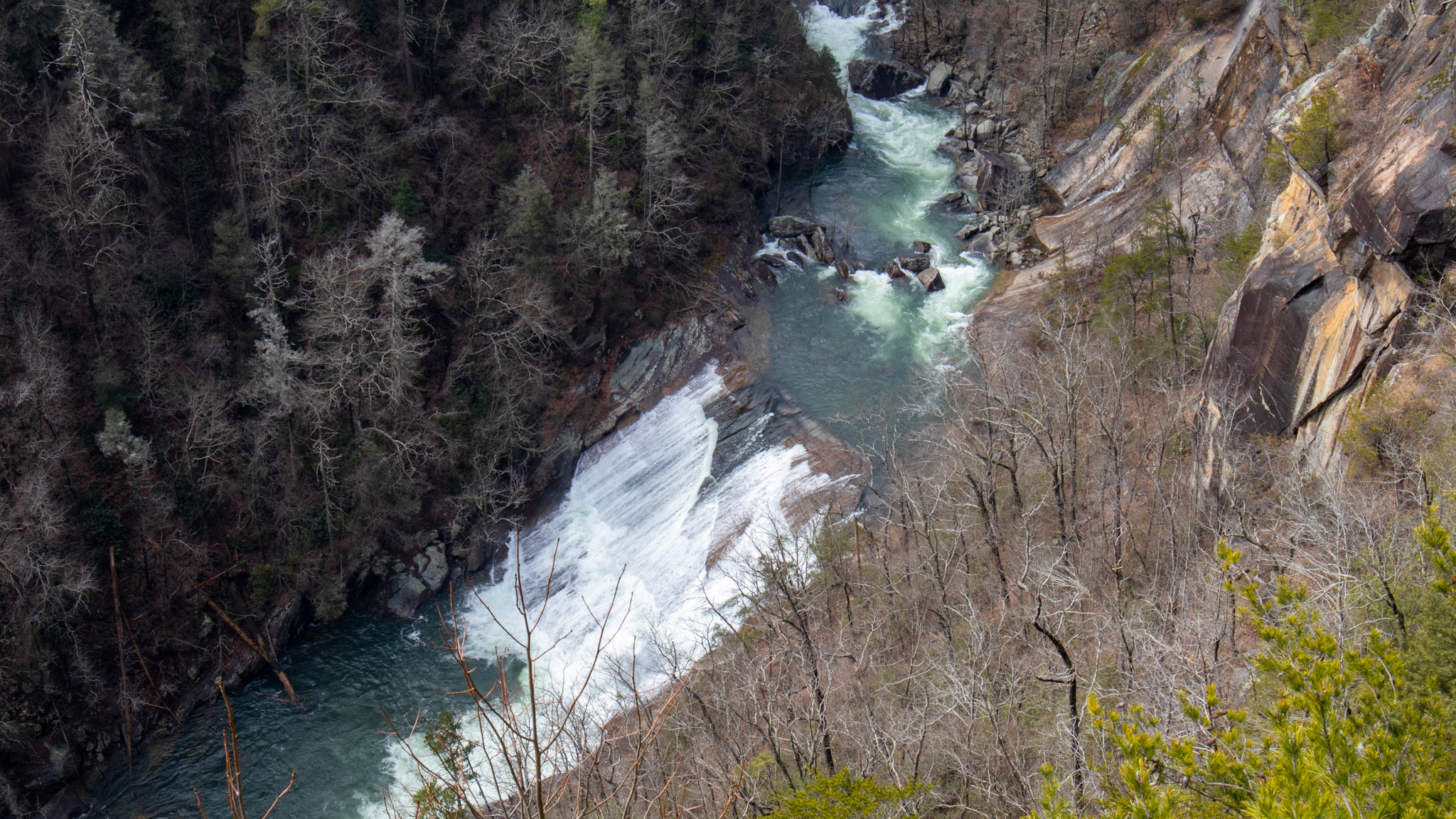
Tallulah Gorge
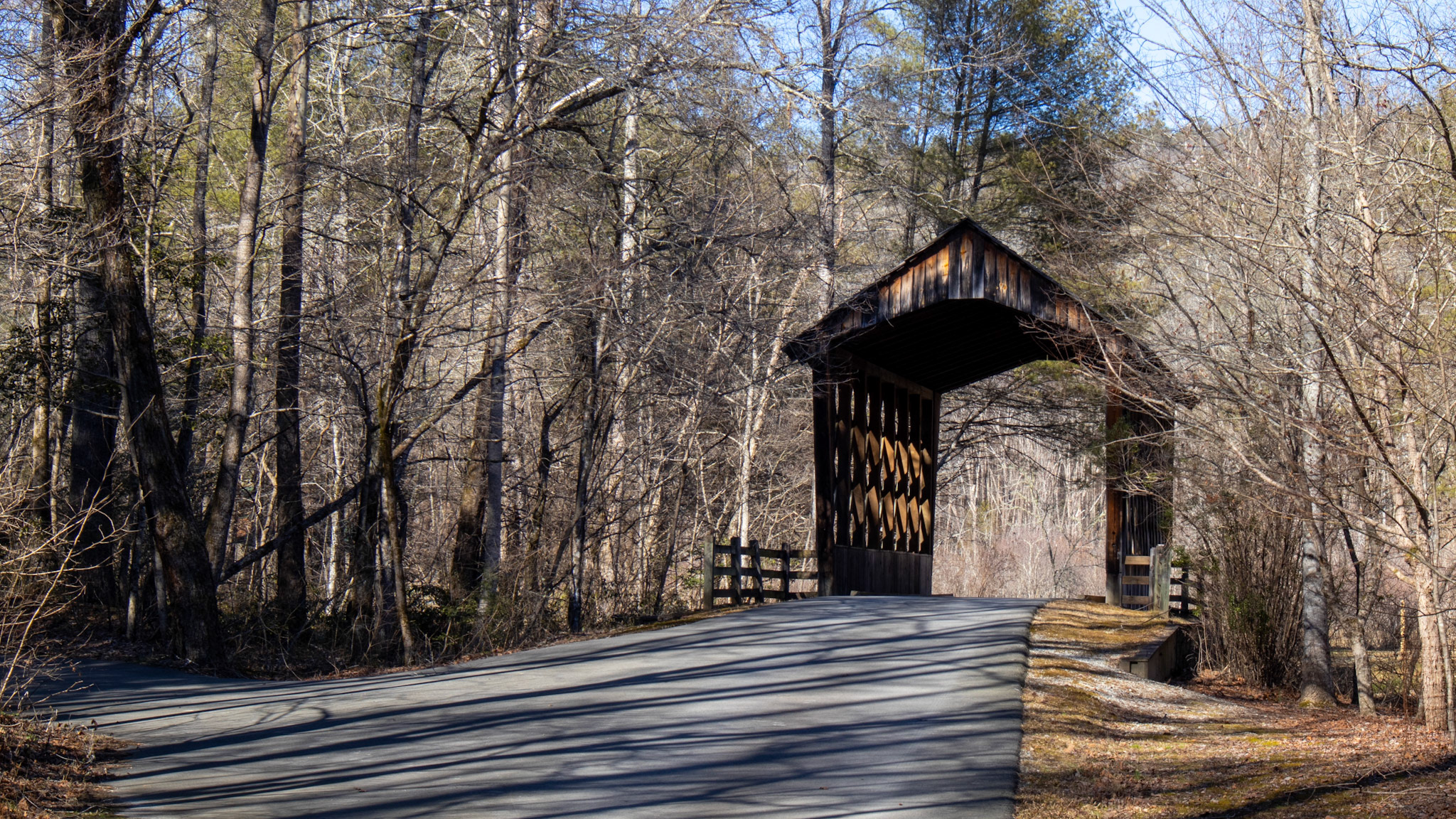
Smithgall Woods
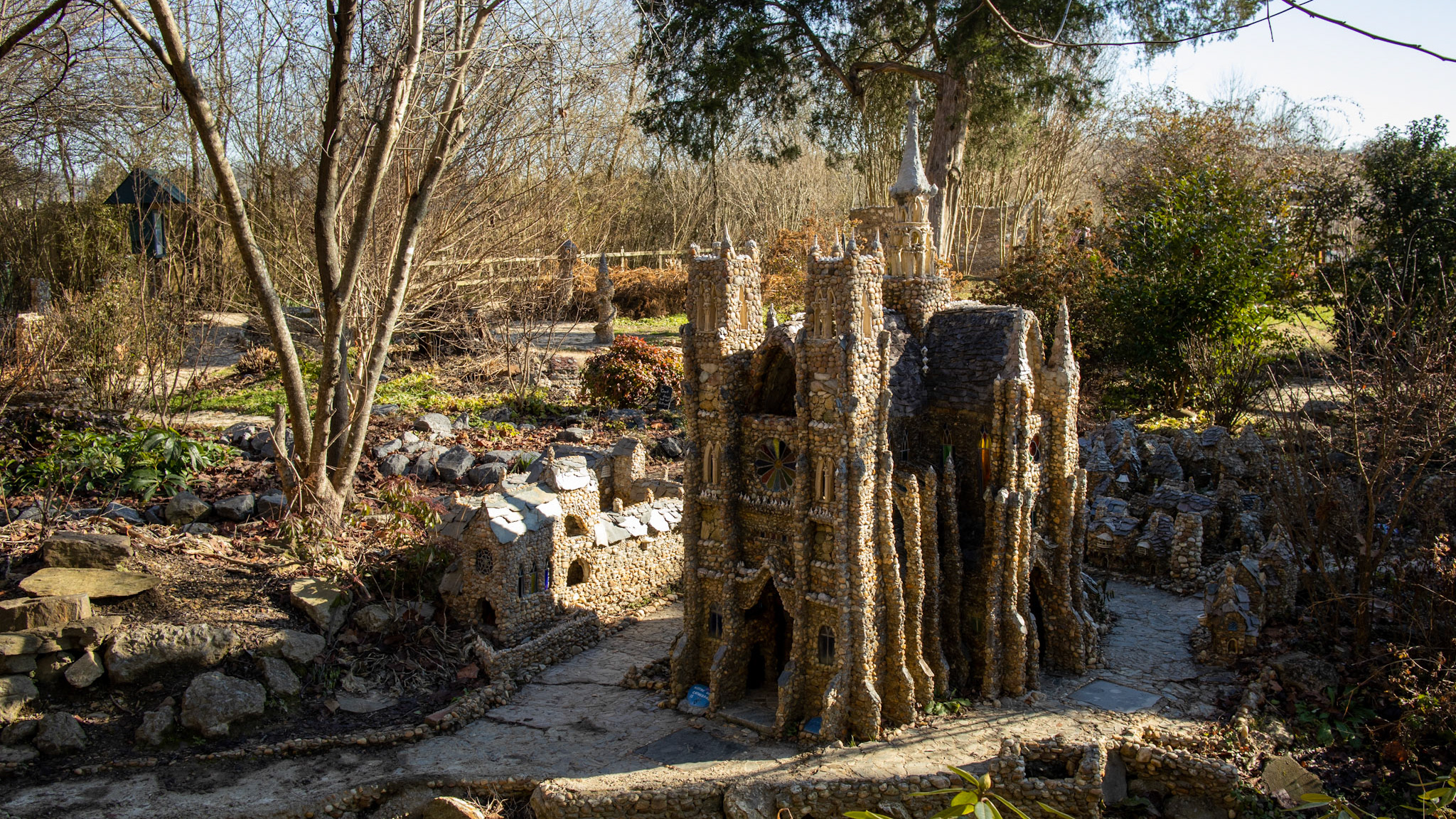
Rock Garden
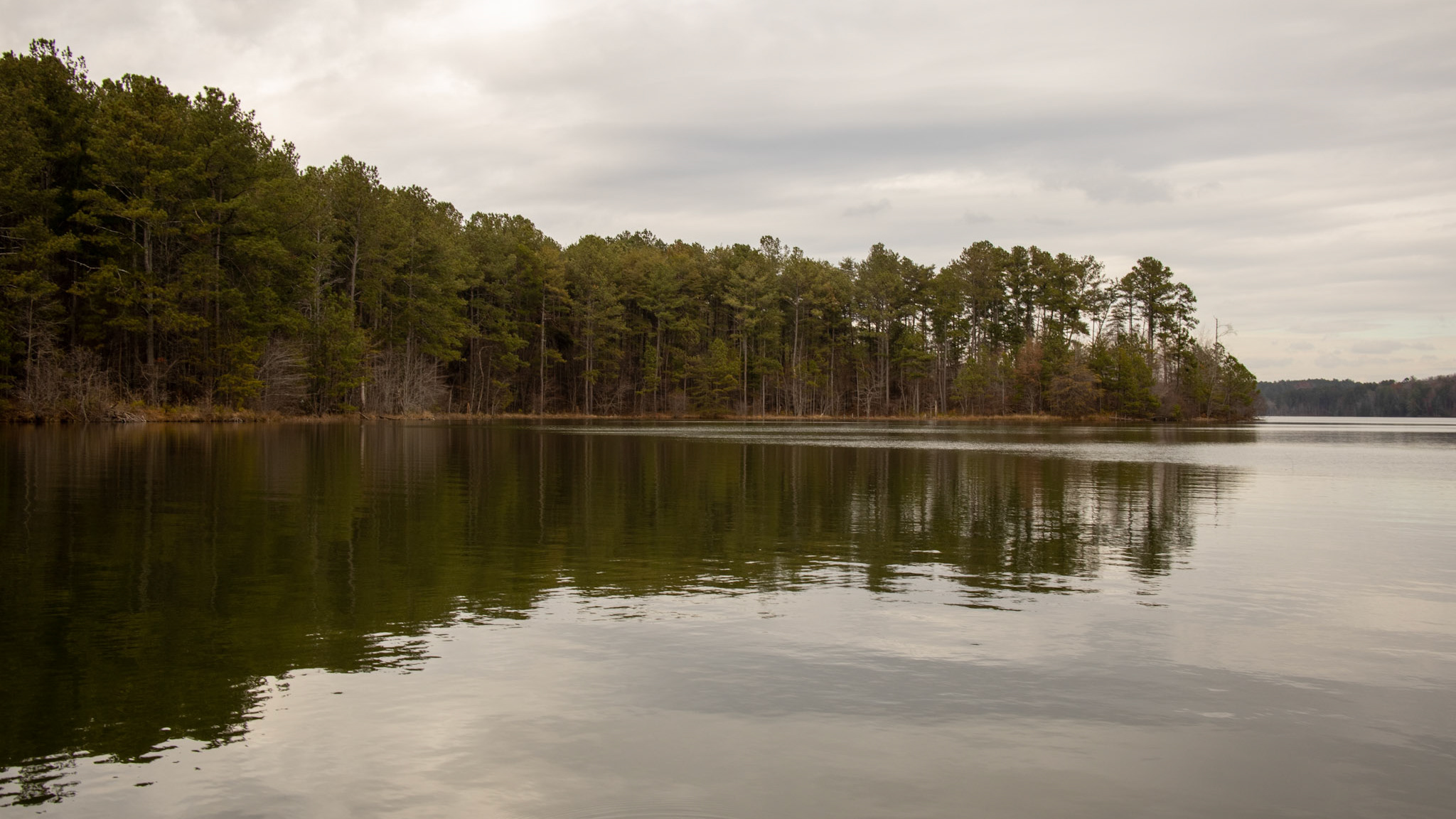
Hollis Q Lathem
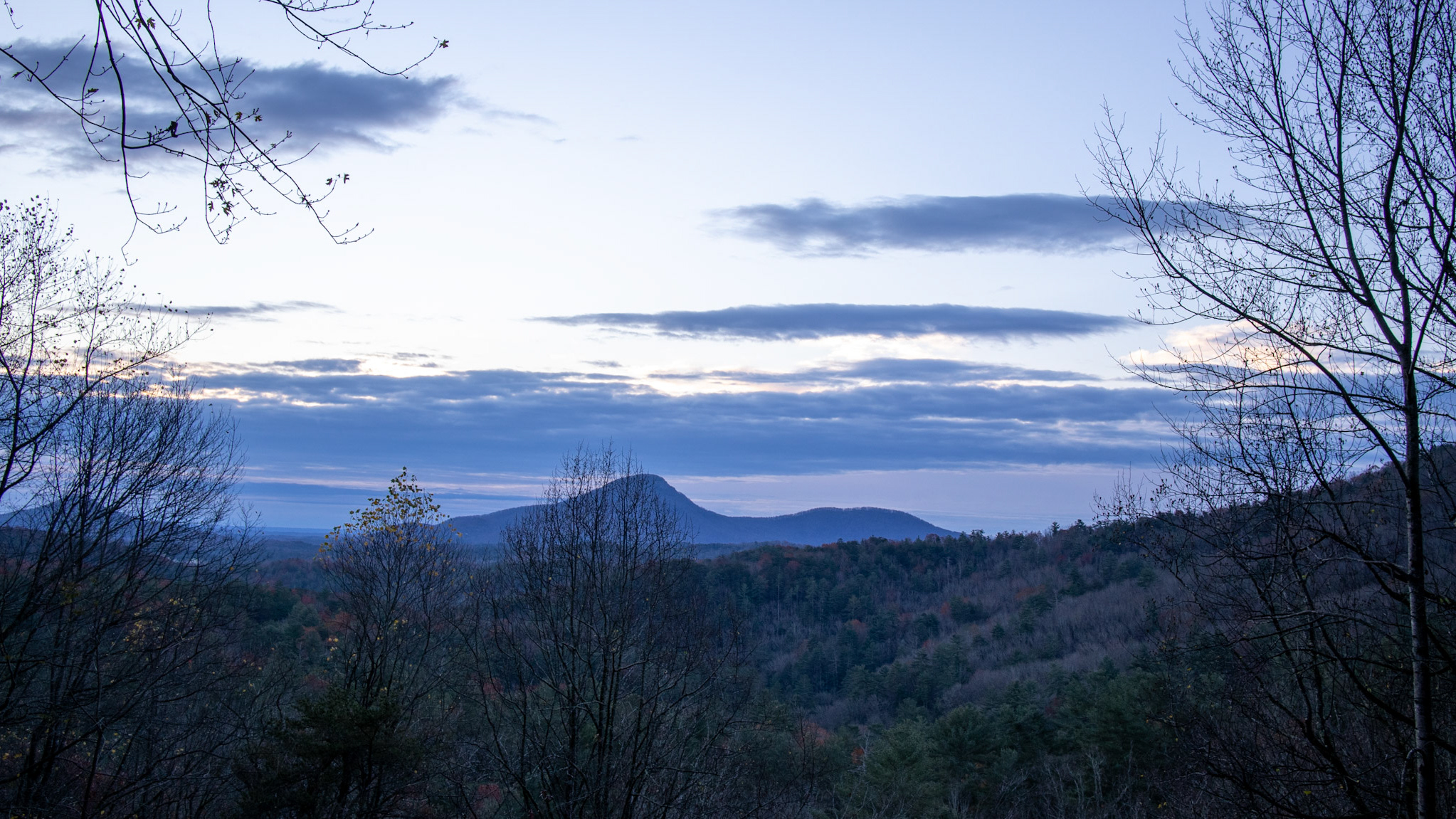
Russell Scenic Hwy
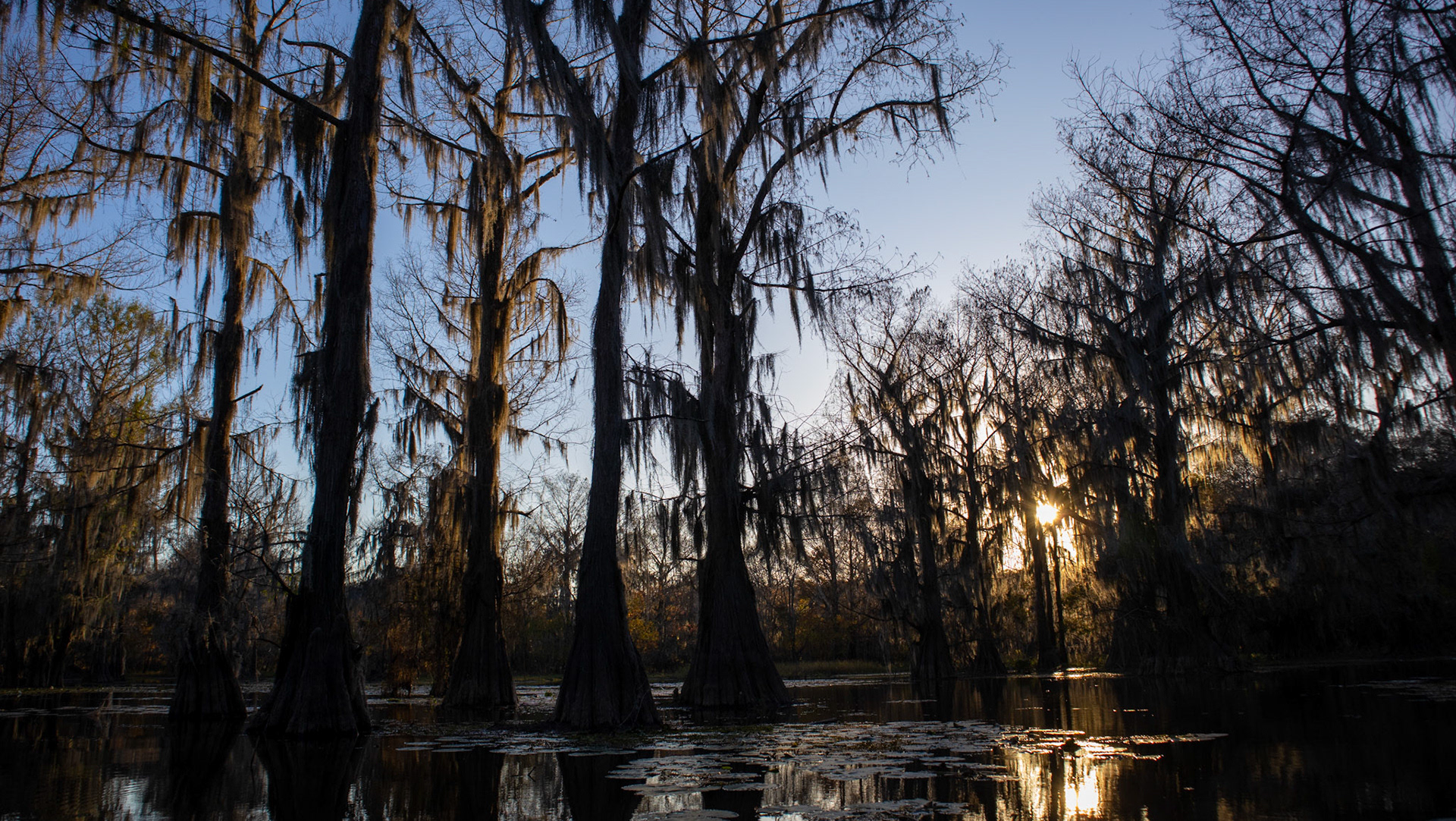
Caddo Lake
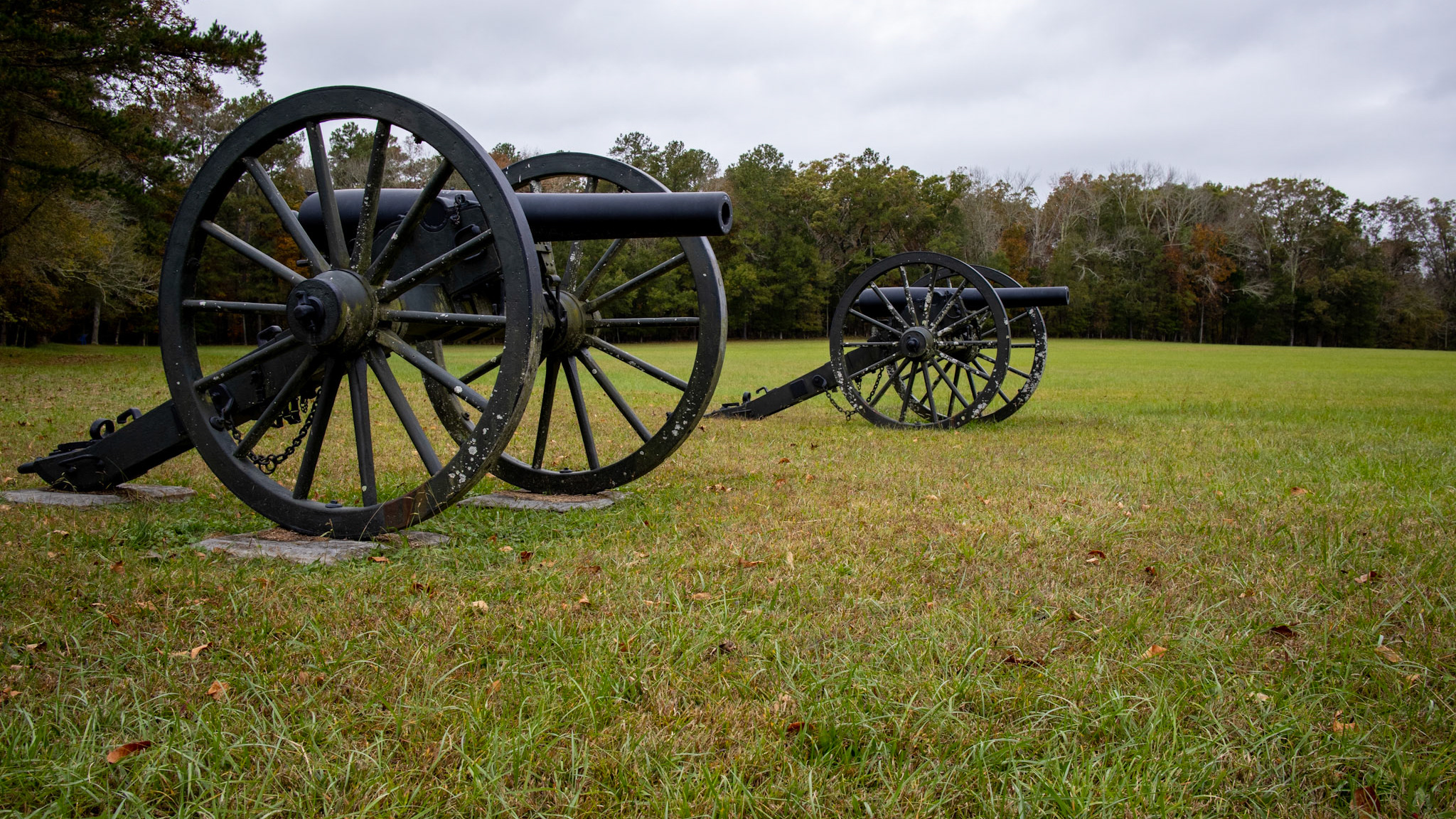
Chickamauga
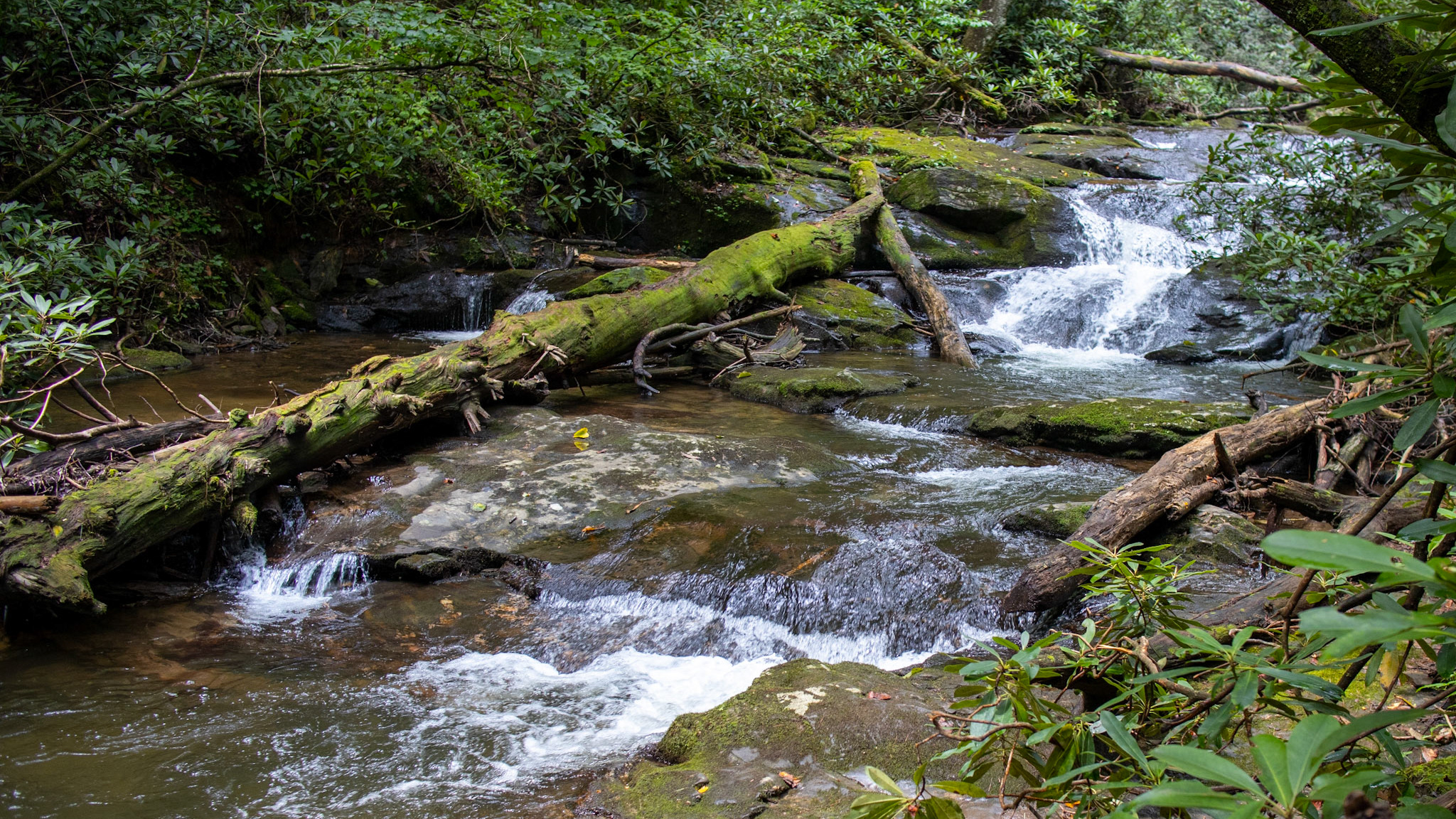
Raven Cliffs
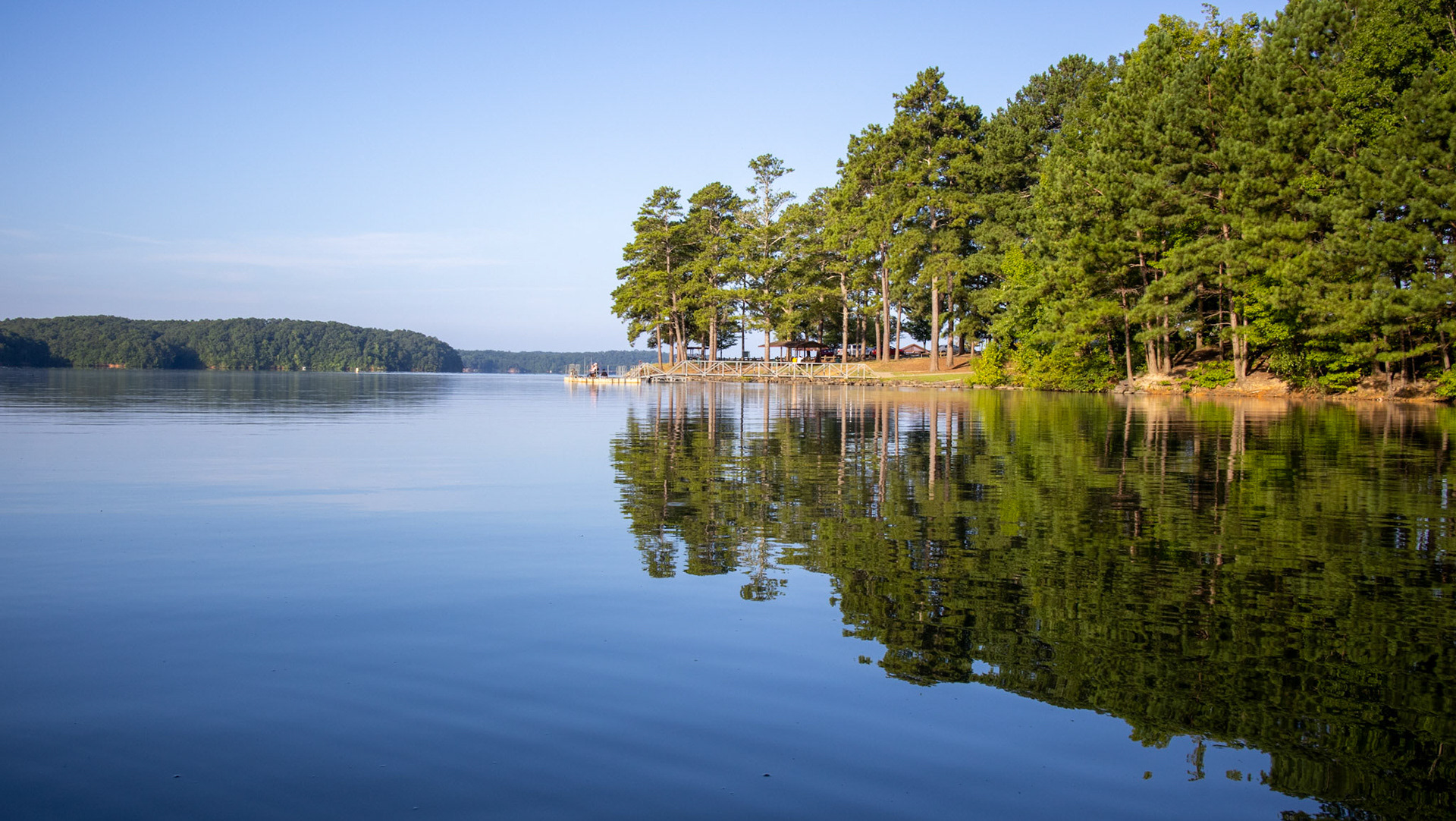
Allatoona
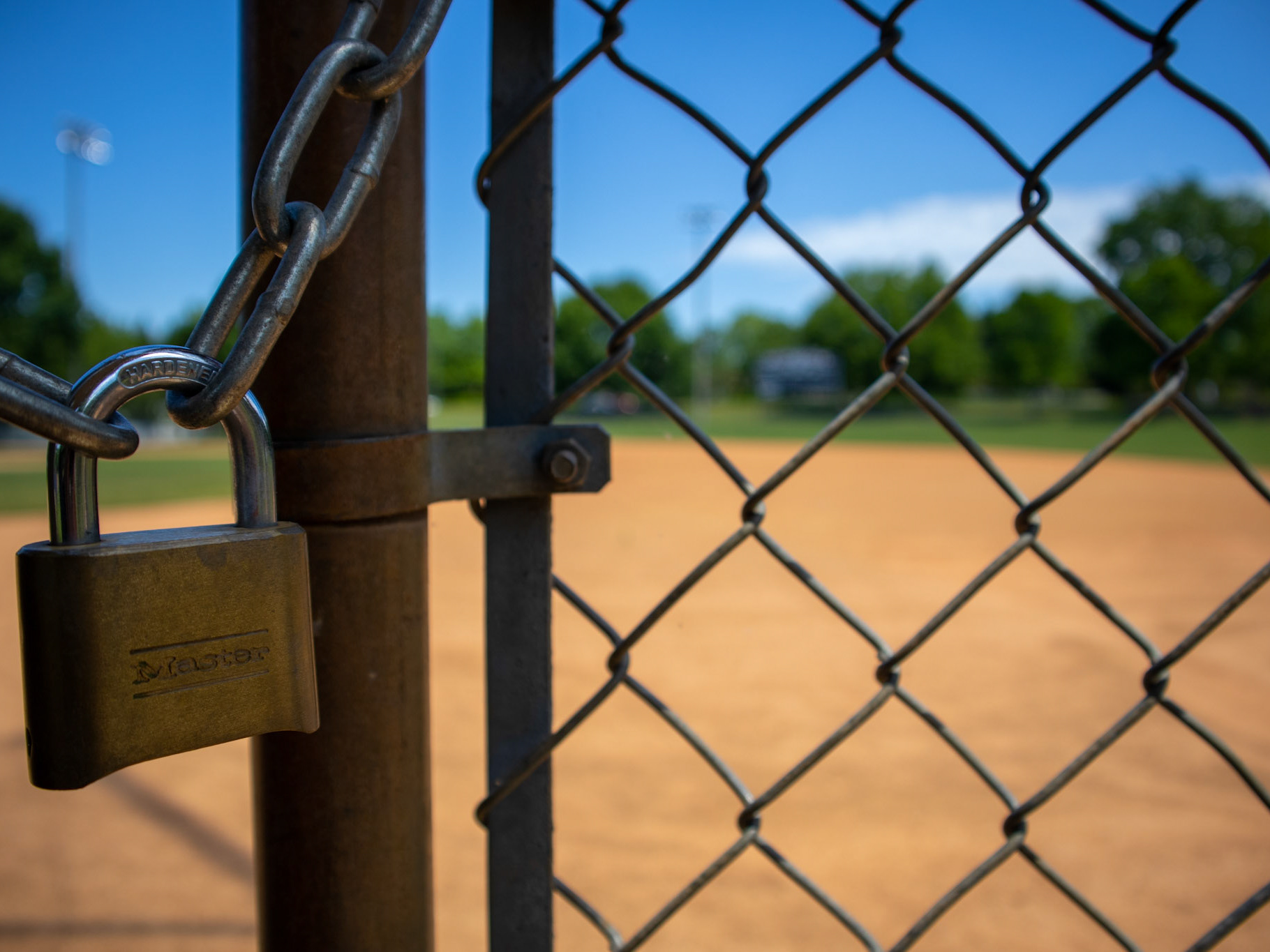
COVID19
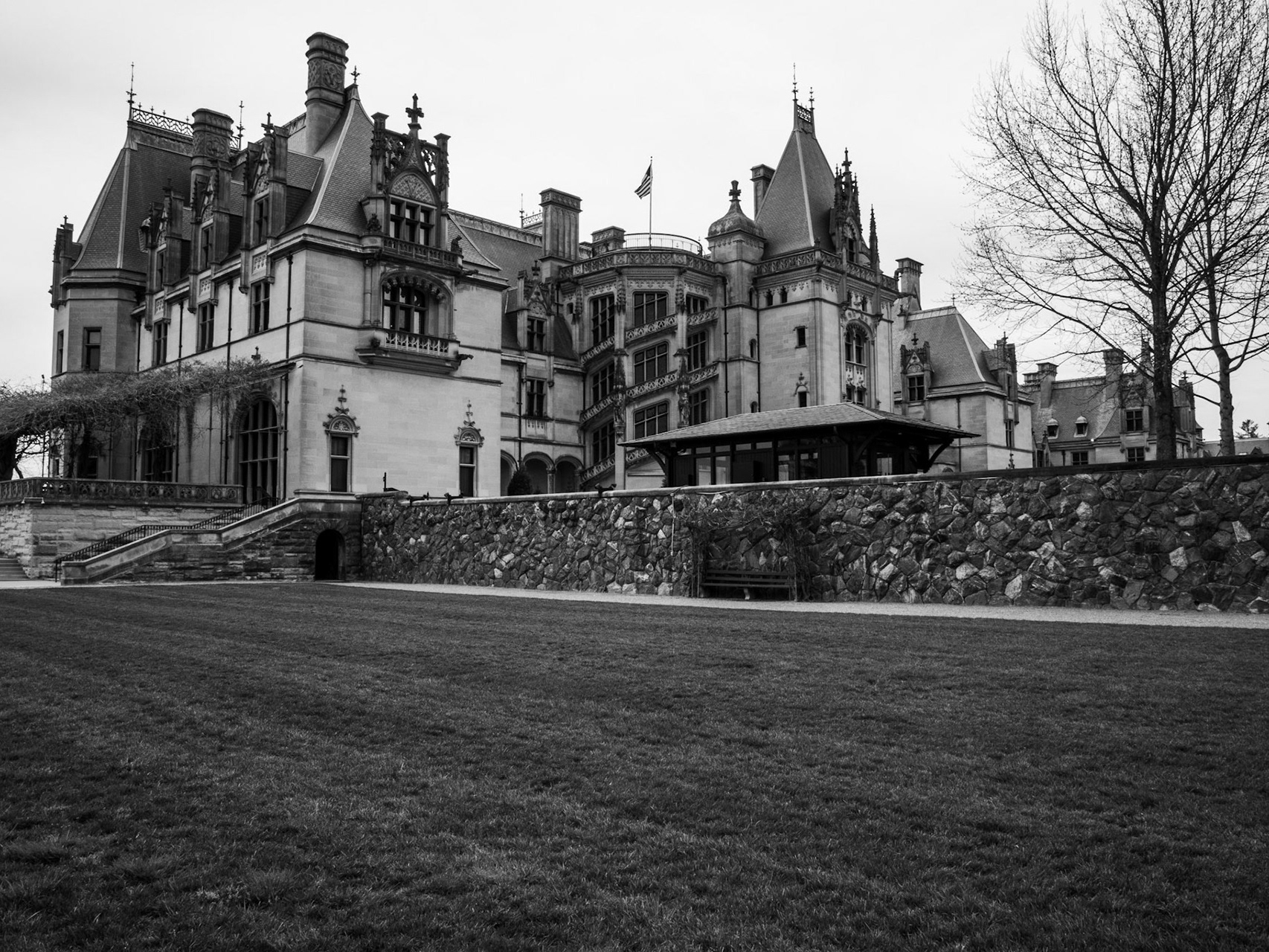
Biltmore
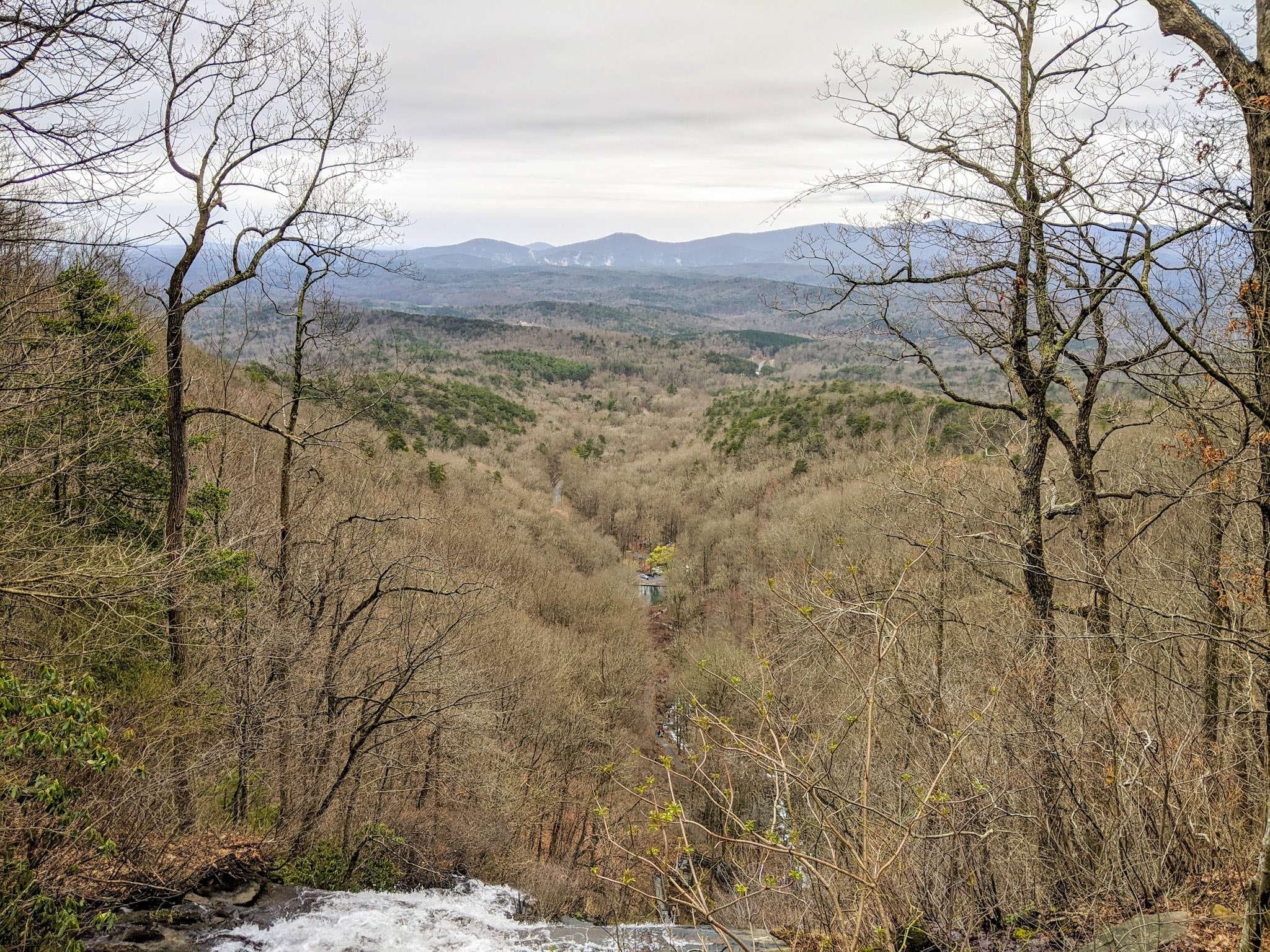
Amicalola Falls
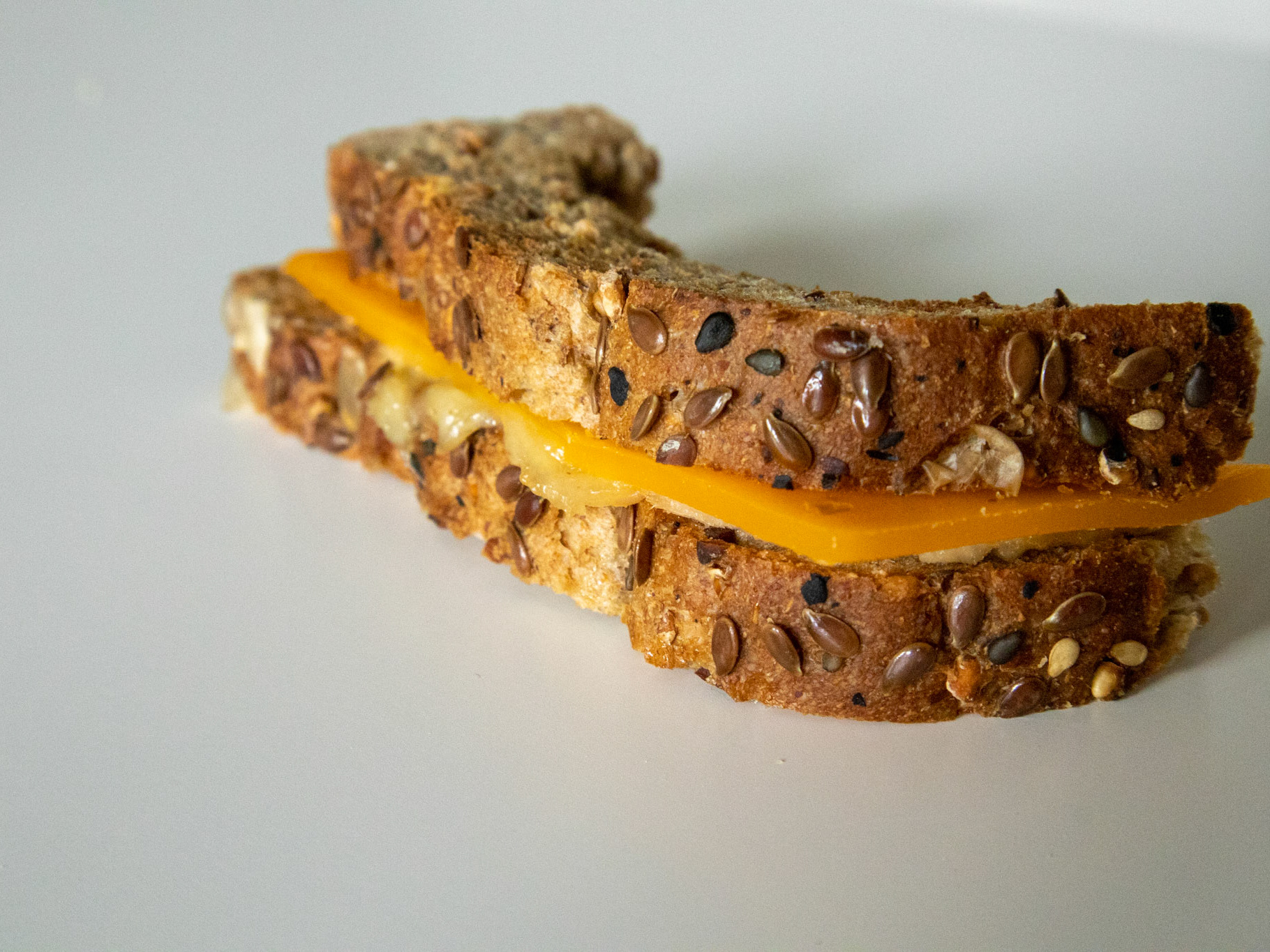
Food
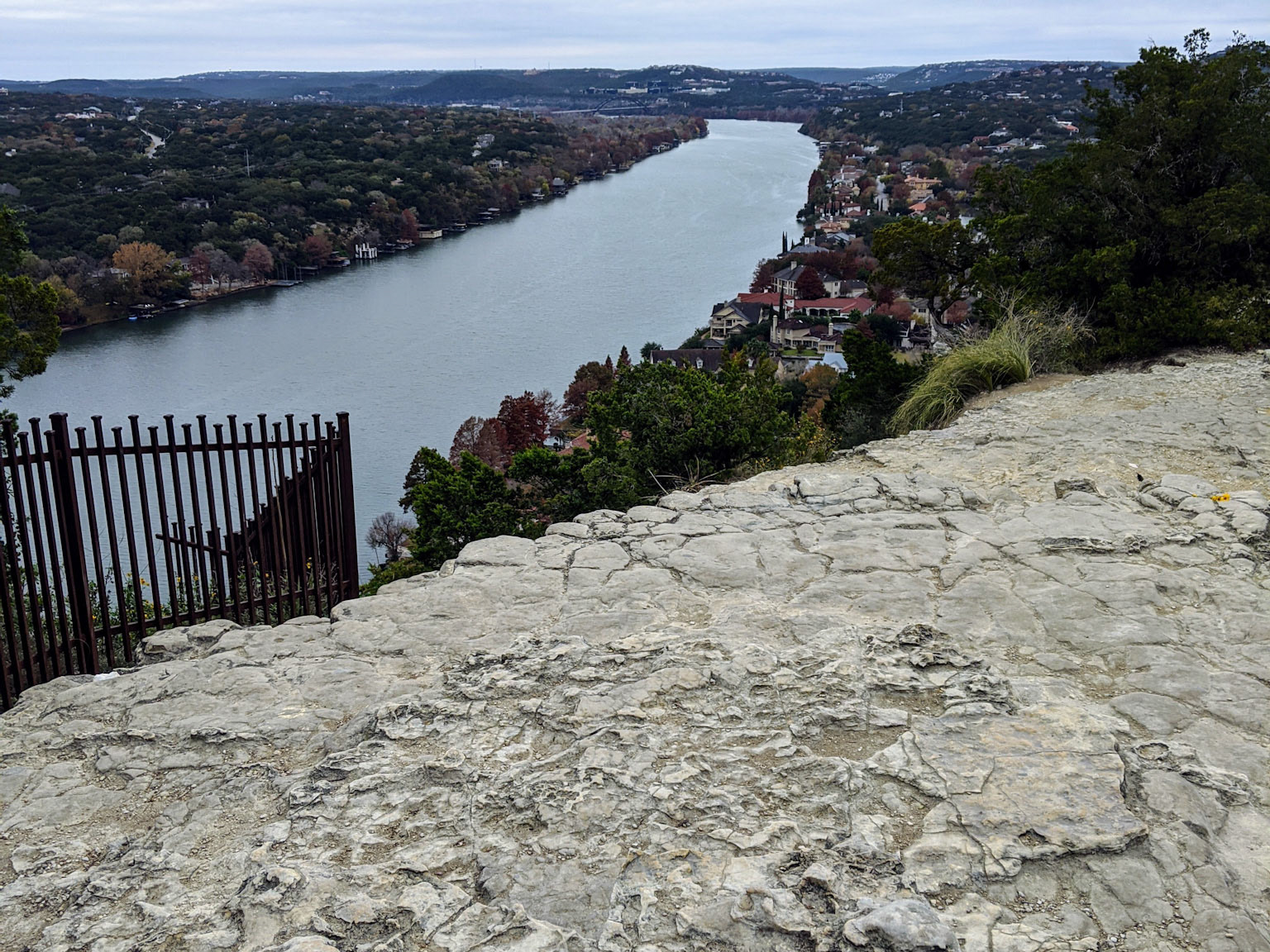
Austin Texas
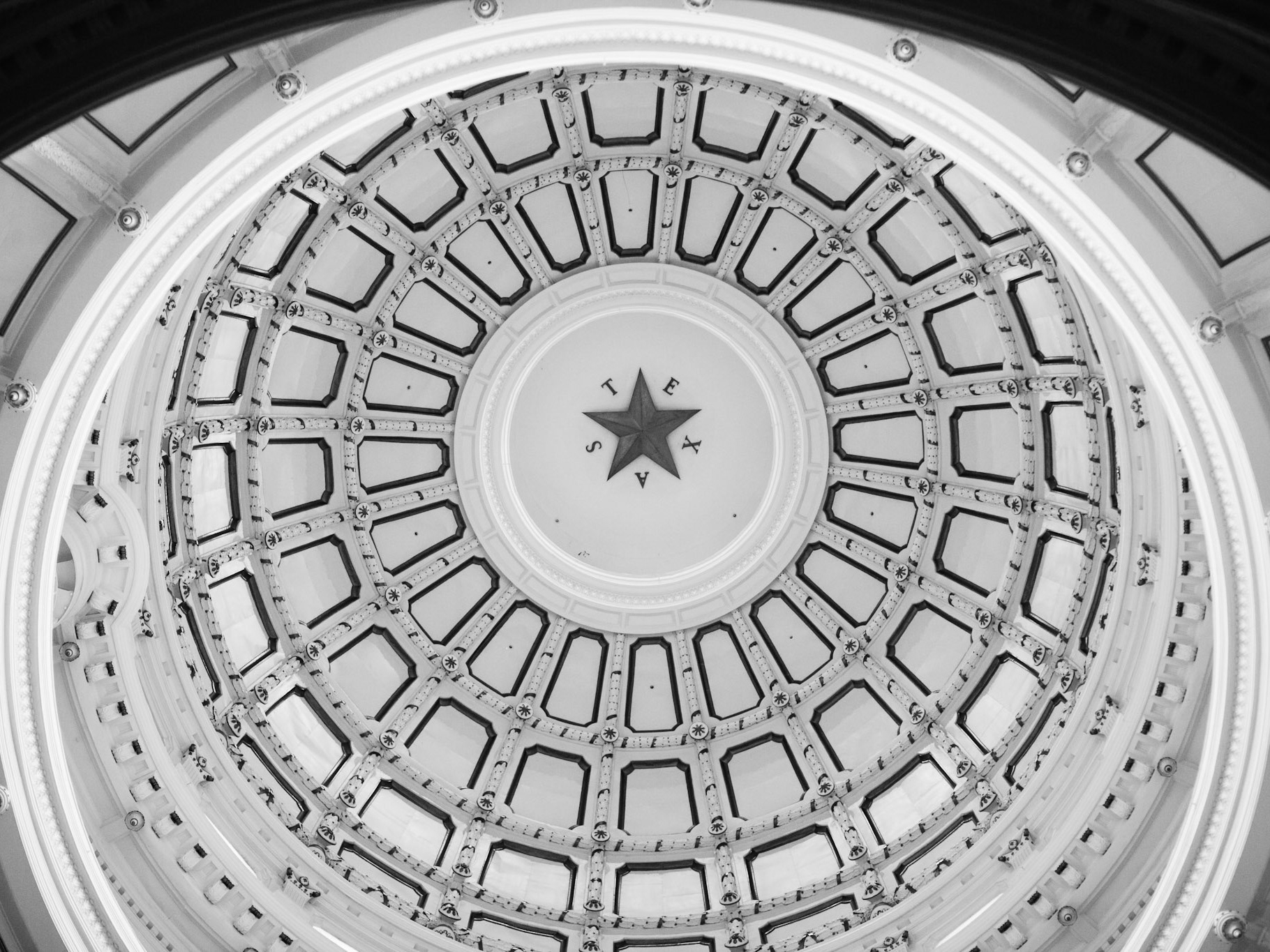
Texas State Capitol